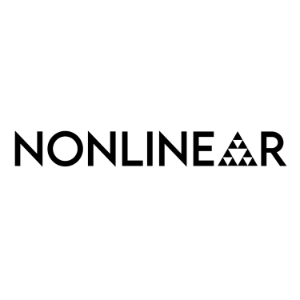
The Nonlinear Library
Education Podcasts
The Nonlinear Library allows you to easily listen to top EA and rationalist content on your podcast player. We use text-to-speech software to create an automatically updating repository of audio content from the EA Forum, Alignment Forum, LessWrong, and other EA blogs. To find out more, please visit us at nonlinear.org
Location:
United States
Genres:
Education Podcasts
Description:
The Nonlinear Library allows you to easily listen to top EA and rationalist content on your podcast player. We use text-to-speech software to create an automatically updating repository of audio content from the EA Forum, Alignment Forum, LessWrong, and other EA blogs. To find out more, please visit us at nonlinear.org
Language:
English
Episodes
LW - CIV: a story by Richard Ngo
6/15/2024
Welcome to The Nonlinear Library, where we use Text-to-Speech software to convert the best writing from the Rationalist and EA communities into audio. This is: CIV: a story, published by Richard Ngo on June 16, 2024 on LessWrong. The room was cozy despite its size, with wood-lined walls reflecting the dim lighting. At one end, a stone fireplace housed a roaring fire; in the middle stood a huge oak table. The woman seated at the head of it rapped her gavel. "I hereby call to order the first meeting of the Parliamentary Subcommittee on Intergalactic Colonization. We'll start with brief opening statements, for which each representative will be allocated one minute, including - " "Oh, enough with the pomp, Victoria. It's just the four of us." The representative for the Liberal Democrats waved his hand around the nearly-empty room. Victoria sniffed. "It's important, Stuart. This is a decision that will have astronomical implications. And it's recorded, besides, so we should do things by the book. Carla, you're up first." The woman at the end of the table stood with a smile. "Thank you, Victoria. I'm speaking on behalf of the Labour party, and I want to start by reminding you all of our place in history. We stand here in a world that has been shaped by centuries of colonialism. Now we're considering another wave of colonization, this one far vaster in scale. We need to - " "Is this just a linguistic argument?" the fourth person at the table drawled. "We can call it something different if that would make you feel better. Say, universe settlement." "Like the settlements in Palestine?" "Oh, come on, Carla." "No, Milton, this is a crucial point. We're talking about the biggest power grab the world has ever seen. You think Leopold II was bad when he was in charge of the Congo? Imagine what people will do if you give each of them total power over a whole solar system! Even libertarians like you have to admit it would be a catastrophe. If there's any possibility that we export oppression from earth across the entire universe, we should burn the rockets and stay home instead." "Okay, thank you Carla," Victoria cut in. "That's time. Stuart, you're up next." Stuart stood. "Speaking on behalf of the Liberal Democrats, I have to admit this is a tricky one. The only feasible way to send humans out to other galaxies is as uploaded minds, but many of our usual principles break for them. I want civilization to be democratic, but what does 'one person one vote' even mean when people can copy and paste themselves? I want human rights for all, but what do human rights even mean when you can just engineer minds who don't want those rights?" "So as much as I hate the idea of segregating civilization, I think it's necessary. Biological humans should get as much territory as we will ever use. But realistically, given the lightspeed constraint, we're never going to actually want to leave the Milky Way. Then the rest of the Virgo Supercluster should be reserved for human uploads. Beyond that, anything else we can reach we should fill with as much happiness and flourishing as possible, no matter how alien it seems to us. After all, as our esteemed predecessor John Stuart Mill once said…" He frowned, and paused for a second. "...as he said, the sole objective of government should be the greatest good for the greatest number." Stuart sat, looking a little disquieted. "Thank you, Stuart. I'll make my opening statement next." Victoria stood and leaned forward, sweeping her eyes across the others. "I'm here representing the Conservatives. It's tempting to think that we can design a good society with just the right social engineering, just the right nudges. But the one thing we conservatives know for sure is: it won't work. Whatever clever plan you come up with, it won't be stable. Given the chance, people will push towards novelty and experimentation and self-modification, and the whole species will end up drifting towards something alien and inhuman. "Hard ru...
Duración:00:15:06
EA - Moral Misdirection (full post) by Richard Y Chappell
6/15/2024
Welcome to The Nonlinear Library, where we use Text-to-Speech software to convert the best writing from the Rationalist and EA communities into audio. This is: Moral Misdirection (full post), published by Richard Y Chappell on June 15, 2024 on The Effective Altruism Forum. I previously included a link to this as part of my trilogy on anti-philanthropic misdirection, but a commenter asked me to post the full text here for the automated audio conversion. This forum post combines my two substack posts on 'Moral Misdirection' and on 'Anti-Philanthropic Misdirection'. Apologies to anyone who has already read them. Moral Misdirection One can lie - or at least misdirect - by telling only truths. Suppose Don shares news of every violent crime committed by immigrants (while ignoring those committed by native-born citizens, and never sharing evidence of immigrants positively contributing to society). He spreads the false impression that immigrants are dangerous and do more harm than good. Since this isn't true, and promulgates harmful xenophobic sentiments, I expect most academics in my social circles would judge Don very negatively, as both (i) morally bad, and (ii) intellectually dishonest. It would not be a convincing defense for Don to say, "But everything I said is literally true!" What matters is that he led his audience to believe much more important falsehoods.[1] I think broadly similar epistemic vices (not always deliberate) are much more common than is generally appreciated. Identifying them requires judgment calls about which truths are most important. These judgment calls are contestable. But I think they're worth making. (Others can always let us know if they think our diagnoses are wrong, which could help to refocus debate on the real crux of the disagreement.) People don't generally think enough about moral prioritization, so encouraging more importance-based criticism could provide helpful correctives against common carelessness and misfocus. Moral misdirection thus strikes me as an important and illuminating concept.[2] In this post, I'll first take an initial stab at clarifying the idea, and then suggest a few examples. (Free free to add more in the comments!) Defining Moral Misdirection Moral misdirection involves leading people morally astray, specifically by manipulating their attention. So explicitly asserting a sincerely believed falsehood doesn't qualify. But misdirection needn't be entirely deliberate, either. Misdirection could be subconscious (perhaps a result of motivated reasoning, or implicit biases), or even entirely inadvertent - merely negligent, say. In fact, deliberately implicating something known to be false won't necessarily count as "misdirection". Innocent examples include simplification, or pedagogical "lies-to-children". If a simplification helps one's audience to better understand what's important, there's nothing dishonest about that - even if it predictably results in some technically false beliefs. Taking all that into account, here's my first stab at a conceptual analysis: Moral misdirection, as it interests me here, is a speech act that functionally operates to distract one's audience from more important moral truths. It thus predictably reduces the importance-weighted accuracy of the audience's moral beliefs. Explanation: Someone who is sincerely, wholeheartedly in error may have the objective effect of leading their audiences astray, but their assertions don't functionally operate towards that end, merely in virtue of happening to be false.[3] Their good-faith erroneous assertions may rather truly aim to improve the importance-weighted accuracy of their audience's beliefs, and simply fail. Mistakes happen. At the other extreme, sometimes people deliberately mislead (about important matters) while technically avoiding any explicit assertion of falsehoods. These bad-faith actors maintain a kind of "plausible deniability" - a sheen of superficial intellectual respectability - while deli...
Duración:00:28:27
LW - MIRI's June 2024 Newsletter by Harlan
6/14/2024
Welcome to The Nonlinear Library, where we use Text-to-Speech software to convert the best writing from the Rationalist and EA communities into audio. This is: MIRI's June 2024 Newsletter, published by Harlan on June 15, 2024 on LessWrong. MIRI updates MIRI Communications Manager Gretta Duleba explains MIRI's current communications strategy. We hope to clearly communicate to policymakers and the general public why there's an urgent need to shut down frontier AI development, and make the case for installing an "off-switch". This will not be easy, and there is a lot of work to be done. Some projects we're currently exploring include a new website, a book, and an online reference resource. Rob Bensinger argues, contra Leopold Aschenbrenner, that the US government should not race to develop artificial superintelligence. "If anyone builds it, everyone dies." Instead, Rob outlines a proposal for the US to spearhead an international alliance to halt progress toward the technology. At the end of June, the Agent Foundations team, including Scott Garrabrant and others, will be parting ways with MIRI to continue their work as independent researchers. The team was originally set up and "sponsored" by Nate Soares and Eliezer Yudkowsky. However, as AI capabilities have progressed rapidly in recent years, Nate and Eliezer have become increasingly pessimistic about this type of work yielding significant results within the relevant timeframes. Consequently, they have shifted their focus to other priorities. Senior MIRI leadership explored various alternatives, including reorienting the Agent Foundations team's focus and transitioning them to an independent group under MIRI fiscal sponsorship with restricted funding, similar to AI Impacts. Ultimately, however, we decided that parting ways made the most sense. The Agent Foundations team has produced some stellar work over the years, and made a true attempt to tackle one of the most crucial challenges humanity faces today. We are deeply grateful for their many years of service and collaboration at MIRI, and we wish them the very best in their future endeavors. The Technical Governance Team responded to NIST's request for comments on draft documents related to the AI Risk Management Framework. The team also sent comments in response to the " Framework for MItigating AI Risks" put forward by U.S. Senators Mitt Romney (R-UT), Jack Reed (D-RI), Jerry Moran (R-KS), and Angus King (I-ME). Brittany Ferrero has joined MIRI's operations team. Previously, she worked on projects such as the Embassy Network and Open Lunar Foundation. We're excited to have her help to execute on our mission. News and links AI alignment researcher Paul Christiano was appointed as head of AI safety at the US AI Safety Institute. Last fall, Christiano published some of his thoughts about AI regulation as well as responsible scaling policies. The Superalignment team at OpenAI has been disbanded following the departure of its co-leaders Ilya Sutskever and Jan Leike. The team was launched last year to try to solve the AI alignment problem in four years. However, Leike says that the team struggled to get the compute it needed and that "safety culture and processes have taken a backseat to shiny products" at OpenAI. This seems extremely concerning from the perspective of evaluating OpenAI's seriousness when it comes to safety and robustness work, particularly given that a similar OpenAI exodus occurred in 2020 in the wake of concerns about OpenAI's commitment to solving the alignment problem. Vox's Kelsey Piper reports that employees who left OpenAI were subject to an extremely restrictive NDA indefinitely preventing them from criticizing the company (or admitting that they were under an NDA), under threat of losing their vested equity in the company. OpenAI executives have since contacted former employees to say that they will not enforce the NDAs. Rob Bensinger comments on these developments here, strongly criticizing OpenAI for...
Duración:00:04:35
LW - Rational Animations' intro to mechanistic interpretability by Writer
6/14/2024
Welcome to The Nonlinear Library, where we use Text-to-Speech software to convert the best writing from the Rationalist and EA communities into audio. This is: Rational Animations' intro to mechanistic interpretability, published by Writer on June 15, 2024 on LessWrong. In our new video, we talk about research on interpreting InceptionV1, a convolutional neural network. Researchers have been able to understand the function of neurons and channels inside the network and uncover visual processing algorithms by looking at the weights. The work on InceptionV1 is early but landmark mechanistic interpretability research, and it functions well as an introduction to the field. We also go into the rationale and goals of the field and mention some more recent research near the end. Our main source material is the circuits thread in the Distill journal and this article on feature visualization. The author of the script is Arthur Frost. I have included the script below, although I recommend watching the video since the script has been written with accompanying moving visuals in mind. Intro In 2018, researchers trained an AI to find out if people were at risk of heart conditions based on pictures of their eyes, and somehow the AI also learned to tell people's biological sex with incredibly high accuracy. How? We're not entirely sure. The crazy thing about Deep Learning is that you can give an AI a set of inputs and outputs, and it will slowly work out for itself what the relationship between them is. We didn't teach AIs how to play chess, go, and atari games by showing them human experts - we taught them how to work it out for themselves. And the issue is, now they have worked it out for themselves, and we don't know what it is they worked out. Current state-of-the-art AIs are huge. Meta's largest LLaMA2 model uses 70 billion parameters spread across 80 layers, all doing different things. It's deep learning models like these which are being used for everything from hiring decisions to healthcare and criminal justice to what youtube videos get recommended. Many experts believe that these models might even one day pose existential risks. So as these automated processes become more widespread and significant, it will really matter that we understand how these models make choices. The good news is, we've got a bit of experience uncovering the mysteries of the universe. We know that humans are made up of trillions of cells, and by investigating those individual cells we've made huge advances in medicine and genetics. And learning the properties of the atoms which make up objects has allowed us to develop modern material science and high-precision technology like computers. If you want to understand a complex system with billions of moving parts, sometimes you have to zoom in. That's exactly what Chris Olah and his team did starting in 2015. They focused on small groups of neurons inside image models, and they were able to find distinct parts responsible for detecting everything from curves and circles to dog heads and cars. In this video we'll Briefly explain how (convolutional) neural networks work Visualise what individual neurons are doing Look at how neurons - the most basic building blocks of the neural network - combine into 'circuits' to perform tasks Explore why interpreting networks is so hard There will also be lots of pictures of dogs, like this one. Let's get going. We'll start with a brief explanation of how convolutional neural networks are built. Here's a network that's trained to label images. An input image comes in on the left, and it flows along through the layers until we get an output on the right - the model's attempt to classify the image into one of the categories. This particular model is called InceptionV1, and the images it's learned to classify are from a massive collection called ImageNet. ImageNet has 1000 different categories of image, like "sandal" and "saxophone" and "sarong" (which, if you don't know, is a k...
Duración:00:16:06
LW - Shard Theory - is it true for humans? by Rishika
6/14/2024
Welcome to The Nonlinear Library, where we use Text-to-Speech software to convert the best writing from the Rationalist and EA communities into audio. This is: Shard Theory - is it true for humans?, published by Rishika on June 14, 2024 on LessWrong. And is it a good model for value learning in AI? TLDR Shard theory proposes a view of value formation where experiences lead to the creation of context-based 'shards' that determine behaviour. Here, we go over psychological and neuroscientific views of learning, and find that while shard theory's emphasis on context bears similarity to types of learning such as conditioning, it does not address top-down influences that may decrease the locality of value-learning in the brain. What's Shard Theory (and why do we care)? In 2022, Quintin Pope and Alex Turner posted ' The shard theory of human values', where they described their view of how experiences shape the value we place on things. They give an example of a baby who enjoys drinking juice, and eventually learns that grabbing at the juice pouch, moving around to find the juice pouch, and modelling where the juice pouch might be, are all helpful steps in order to get to its reward. 'Human values', they say, 'are not e.g. an incredibly complicated, genetically hard-coded set of drives, but rather sets of contextually activated heuristics…' And since, like humans, AI is often trained with reinforcement learning, the same might apply to AI. The original post is long (over 7,000 words) and dense, but Lawrence Chan helpfully posted a condensation of the topic in ' Shard Theory in Nine Theses: a Distillation and Critical Appraisal'. In it, he presents nine (as might be expected) main points of shard theory, ending with the last thesis: 'shard theory as a model of human values'. 'I'm personally not super well versed in neuroscience or psychology', he says, 'so I can't personally attest to [its] solidity…I'd be interested in hearing from experts in these fields on this topic.' And that's exactly what we're here to do. A Crash Course on Human Learning Types of learning What is learning? A baby comes into the world and is inundated with sensory information of all kinds. From then on, it must process this information, take whatever's useful, and store it somehow for future use. There's various places in the brain where this information is stored, and for various purposes. Looking at these various types of storage, or memory, can help us understand what's going on: 3 types of memory We often group memory types by the length of time we hold on to them - 'working memory' (while you do some task), 'short-term memory' (maybe a few days, unless you revise or are reminded), and 'long-term memory' (effectively forever). Let's take a closer look at long-term memory: Types of long-term memory We can broadly split long-term memory into 'declarative' and 'nondeclarative'. Declarative memory is stuff you can talk about (or 'declare'): what the capital of your country is, what you ate for lunch yesterday, what made you read this essay. Nondeclarative covers the rest: a grab-bag of memory types including knowing how to ride a bike, getting habituated to a scent you've been smelling all day, and being motivated to do things you were previously rewarded for (like drinking sweet juice). For most of this essay, we'll be focusing on the last type: conditioning. Types of conditioning Conditioning Sometime in the 1890s, a physiologist named Ivan Pavlov was researching salivation using dogs. He would feed the dogs with powdered meat, and insert a tube into the cheek of each dog to measure their saliva.As expected, the dogs salivated when the food was in front of them. Unexpectedly, the dogs also salivated when they heard the footsteps of his assistant (who brought them their food). Fascinated by this, Pavlov started to play a metronome whenever he gave the dogs their food. After a while, sure enough, the dogs would salivate whenever the metronome played, even if ...
Duración:00:27:05
EA - What "Effective Altruism" Means to Me by Richard Y Chappell
6/14/2024
Welcome to The Nonlinear Library, where we use Text-to-Speech software to convert the best writing from the Rationalist and EA communities into audio. This is: What "Effective Altruism" Means to Me, published by Richard Y Chappell on June 14, 2024 on The Effective Altruism Forum. I previously included a link to this as part of my trilogy on anti-philanthropic misdirection, but a commenter asked me to post the full text here for the automated audio conversion. Apologies to anyone who has already read it. As I wrote in 'Why Not Effective Altruism?', I find the extreme hostility towards effective altruism from some quarters to be rather baffling. Group evaluations can be vexing: perhaps what the critics have in mind when they hate on EA has little or no overlap with what I have in mind when I support it? It's hard to know without getting into details, which the critics rarely do. So here are some concrete claims that I think are true and important. If you disagree with any of them, I'd be curious to hear which ones, and why! What I think: 1. It's good and virtuous to be beneficent and want to help others, for example by taking the Giving What We Can 10% pledge. 2. It's good and virtuous to want to help others effectively: to help more rather than less with one's efforts. 3. We have the potential to do a lot of good in the face of severe global problems (including global poverty, factory-farmed animal welfare, and protecting against global catastrophic risks such as future pandemics). 4. In all these areas, it is worth making deliberate, informed efforts to act effectively. Better targeting our efforts may make even more of a difference than the initial decision to help at all. 5. In all these areas, we can find interventions that we can reasonably be confident are very positive in expectation. (One can never be so confident of actual outcomes in any given instance, but being robustly positive in prospect is what's decision-relevant.) 6. Beneficent efforts can be expected to prove (much) more effective if guided by careful, in-depth empirical research. Quantitative tools and evidence, used wisely, can help us to do more good. 7. So it's good and virtuous to use quantitatively tools and evidence wisely. 8. GiveWell does incredibly careful, in-depth empirical research evaluating promising-seeming global charities, using quantitative tools and evidence wisely. 9. So it's good and virtuous to be guided by GiveWell (or comparably high-quality evaluators) rather than less-effective alternatives like choosing charities based on locality, personal passion, or gut feelings. 10. There's no good reason to think that GiveWell's top charities are net harmful.[1] 11. But even if you're the world's most extreme aid skeptic, it's clearly good and virtuous to voluntary redistribute your own wealth to some of the world's poorest people via GiveDirectly. (And again: more good and virtuous than typical alternatives.) 12. Many are repelled by how "hands-off" effective philanthropy is compared to (e.g.) local volunteering. But it's good and virtuous to care more about saving and improving lives than about being hands on. To prioritize the latter over the former would be morally self-indulgent. 13. Hits-based giving is a good idea. A portfolio of long shots can collectively be likely to do more good than putting all your resources into lower-expected-value "sure things". In such cases, this is worth doing. 14. Even if one-off cases, it is often better and more virtuous to accept some risk of inefficacy in exchange for a reasonable shot at proportionately greater positive impact. (But reasonable people can disagree about which trade-offs of this sort are worth it.) 15. The above point encompasses much relating to politics and "systemic change", in addition to longtermist long-shots. It's very possible for well-targeted efforts in these areas to be even better in expectation than traditional philanthropy - just note that this potential impact comes at ...
Duración:00:13:24
EA - Help Fund Insect Welfare Science by Bob Fischer
6/14/2024
Welcome to The Nonlinear Library, where we use Text-to-Speech software to convert the best writing from the Rationalist and EA communities into audio. This is: Help Fund Insect Welfare Science, published by Bob Fischer on June 14, 2024 on The Effective Altruism Forum. The Arthropoda Foundation Tens of trillions of insects are used or killed by humans across dozens of industries. Despite being the most numerous animal species reared by animal industries, we know next to nothing about what's good or bad for these animals. And right now, funding for this work is scarce. Traditional science funders won't pay for it; and within EA, the focus is on advocacy, not research. So, welfare science needs your help. We're launching the Arthropoda Foundation, a fund to ensure that insect welfare science gets the essential resources it needs to provide decision-relevant answers to pressing questions. Every dollar we raise will be granted to research projects that can't be funded any other way. We're in a critical moment for this work. Over the last year, field-building efforts have accelerated, setting up academic labs that can tackle key studies. However, funding for these studies is now uncertain. We need resources to sustain the research required to improve the welfare of insects. Why do we need a fund? We need a fund because we need a runway for high-priority research. Scientists need to make plans over several years, not a few months. They have to commit now to a grad student who starts next year and finishes a project two years after that. The fund helps guarantee that resources will be there to support academics in the long-term, ensuring that entire labs can remain devoted to this work. We need a fund because we need to let researchers be researchers, not fundraisers. A fund doesn't just buy critical research; it buys the ability of the world's few insect welfare scientists to focus on what matters. We need a fund because funding scientific research on insect welfare isn't easy for individual donors. First, it's hard to know what to fund. As some of the few researchers who have worked on these issues in EA, we're lending our expertise to vet opportunities. Second, universities take overhead that reduces the impact of your donations; an independent fund can use the board's volunteer labor to make the many small reimbursements that are required to cover costs directly. Third, if you're a donor who's giving below the amounts required to support entire projects, your opportunities are extremely limited. This fund smooths over such hurdles, ensuring that everyone can support the highest value research. This fund gives a brand new field some time to get established, it gives that field the resources required to produce essential science, and it keeps that research as cost-effective as possible. Please support welfare science. Team Bob Fischer is a Professor at Texas State University and the lead project manager and author of the Moral Weight Project, a research project to build comparative models of moral weight across animal species. Daniela Waldhorn is the Director of Animal Welfare research at Rethink Priorities, a board member of the Centre for Animal Ethics at Pompeu Fabra University, and lead author on the largest initial EA project focused on studying invertebrate welfare. Abraham Rowe is the Principal of Good Structures, a nonprofit operations service provider, and was previously the COO of Rethink Priorities, and the co-founder and Executive Director of Wild Animal Initiative, an academic field-building and grantmaking organization supporting research on wild animal welfare. Thanks for listening. To help us out with The Nonlinear Library or to learn more, please visit nonlinear.org
Duración:00:03:23
EA - [Linkpost] An update from Good Ventures by Alexander Berger
6/14/2024
Welcome to The Nonlinear Library, where we use Text-to-Speech software to convert the best writing from the Rationalist and EA communities into audio. This is: [Linkpost] An update from Good Ventures, published by Alexander Berger on June 14, 2024 on The Effective Altruism Forum. I wanted to share this update from Good Ventures (Cari and Dustin's philanthropy), which seems relevant to the EA community. Tl;dr: "while we generally plan to continue increasing our grantmaking in our existing focus areas via our partner Open Philanthropy, we have decided to exit a handful of sub-causes (amounting to less than 5% of our annual grantmaking), and we are no longer planning to expand into new causes in the near term by default." A few follow-ups on this from an Open Phil perspective: I want to apologize to directly affected grantees (who've already been notified) for the negative surprise here, and for our part in not better anticipating it. While this represents a real update, we remain deeply aligned with Good Ventures (they're expecting to continue to increase giving via OP over time), and grateful for how many of the diverse funding opportunities we've recommended that they've been willing to tackle. An example of a new potential focus area that OP staff had been interested in exploring that Good Ventures is not planning to fund is research on the potential moral patienthood of digital minds. If any readers are interested in funding opportunities in that space, please reach out. Good Ventures has told us they don't plan to exit any overall focus areas in the near term. But this update is an important reminder that such a high degree of reliance on one funder (especially on the GCR side) represents a structural risk. I think it's important to diversify funding in many of the fields Good Ventures currently funds, and that doing so could make the funding base more stable both directly (by diversifying funding sources) and indirectly (by lowering the time and energy costs to Good Ventures from being such a disproportionately large funder). Another implication of these changes is that going forward, OP will have a higher bar for recommending grants that could draw on limited Good Ventures bandwidth, and so our program staff will face more constraints in terms of what they're able to fund. We always knew we weren't funding every worthy thing out there, but that will be even more true going forward. Accordingly, we expect marginal opportunities for other funders to look stronger going forward. Historically, OP has been focused on finding enough outstanding giving opportunities to hit Good Ventures' spending targets, with a long-term vision that once we had hit those targets, we'd expand our work to support other donors seeking to maximize their impact. We'd already gotten a lot closer to GV's spending targets over the last couple of years, but this update has accelerated our timeline for investing more in partnerships and advising other philanthropists. If you're interested, please consider applying or referring candidates to lead our new partnerships function. And if you happen to be a philanthropist looking for advice on how to invest >$1M/year in new cause areas, please get in touch. Thanks for listening. To help us out with The Nonlinear Library or to learn more, please visit nonlinear.org
Duración:00:02:54
AF - Shard Theory - is it true for humans? by ErisApprentice
6/14/2024
Welcome to The Nonlinear Library, where we use Text-to-Speech software to convert the best writing from the Rationalist and EA communities into audio. This is: Shard Theory - is it true for humans?, published by ErisApprentice on June 14, 2024 on The AI Alignment Forum. And is it a good model for value learning in AI? (Read on Substack: https://recursingreflections.substack.com/p/shard-theory-is-it-true-for-humans) TLDR Shard theory proposes a view of value formation where experiences lead to the creation of context-based 'shards' that determine behaviour. Here, we go over psychological and neuroscientific views of learning, and find that while shard theory's emphasis on context bears similarity to types of learning such as conditioning, it does not address top-down influences that may decrease the locality of value-learning in the brain. What's Shard Theory (and why do we care)? In 2022, Quintin Pope and Alex Turner posted ' The shard theory of human values', where they described their view of how experiences shape the value we place on things. They give an example of a baby who enjoys drinking juice, and eventually learns that grabbing at the juice pouch, moving around to find the juice pouch, and modelling where the juice pouch might be, are all helpful steps in order to get to its reward. 'Human values', they say, 'are not e.g. an incredibly complicated, genetically hard-coded set of drives, but rather sets of contextually activated heuristics…' And since, like humans, AI is often trained with reinforcement learning, the same might apply to AI. The original post is long (over 7,000 words) and dense, but Lawrence Chan helpfully posted a condensation of the topic in ' Shard Theory in Nine Theses: a Distillation and Critical Appraisal'. In it, he presents nine (as might be expected) main points of shard theory, ending with the last thesis: 'shard theory as a model of human values'. 'I'm personally not super well versed in neuroscience or psychology', he says, 'so I can't personally attest to [its] solidity…I'd be interested in hearing from experts in these fields on this topic.' And that's exactly what we're here to do. A Crash Course on Human Learning Types of learning What is learning? A baby comes into the world and is inundated with sensory information of all kinds. From then on, it must process this information, take whatever's useful, and store it somehow for future use. There's various places in the brain where this information is stored, and for various purposes. Looking at these various types of storage, or memory, can help us understand what's going on: 3 types of memory We often group memory types by the length of time we hold on to them - 'working memory' (while you do some task), 'short-term memory' (maybe a few days, unless you revise or are reminded), and 'long-term memory' (effectively forever). Let's take a closer look at long-term memory: Types of long-term memory We can broadly split long-term memory into 'declarative' and 'nondeclarative'. Declarative memory is stuff you can talk about (or 'declare'): what the capital of your country is, what you ate for lunch yesterday, what made you read this essay. Nondeclarative covers the rest: a grab-bag of memory types including knowing how to ride a bike, getting habituated to a scent you've been smelling all day, and being motivated to do things you were previously rewarded for (like drinking sweet juice). For most of this essay, we'll be focusing on the last type: conditioning. Types of conditioning Conditioning Sometime in the 1890s, a physiologist named Ivan Pavlov was researching salivation using dogs. He would feed the dogs with powdered meat, and insert a tube into the cheek of each dog to measure their saliva.As expected, the dogs salivated when the food was in front of them. Unexpectedly, the dogs also salivated when they heard the footsteps of his assistant (who brought them their food). Fascinated by this, Pavlov started to play a metronome whenever h...
Duración:00:27:18
AF - Fine-tuning is not sufficient for capability elicitation by Theodore Chapman
6/14/2024
Welcome to The Nonlinear Library, where we use Text-to-Speech software to convert the best writing from the Rationalist and EA communities into audio. This is: Fine-tuning is not sufficient for capability elicitation, published by Theodore Chapman on June 14, 2024 on The AI Alignment Forum. Produced as part of the ML Alignment & Theory Scholars Program - Winter 2023-24 Cohort under the supervision of Evan Hubinger. Acknowledgements: Thanks to Kyle Brady for his many contributions to this project. Abstract This post argues that the performance elicited by fine-tuning an LLM on a task using a given prompt format does not usefully bound the level of performance observed when the same information is presented in a different structure. Thus, fine-tuned performance provides very little information about the best performance that would be achieved by a large number of actors fine-tuning models with random prompting schemes in parallel. In particular, we find that we get much better results from fine-tuning gpt-3.5-turbo (ChatGPT 3.5) to play chess when the game so far is presented in a single block of SAN[1] than when the game so far is separated into a series of SAN moves presented as alternating user / assistant messages. The fact that this superficial formatting change is sufficient to change our fine-tuned performance serves to highlight that modern LLMs are much more fragile than they appear at first glance, even subject to fine-tuning. Introduction In the abstract, model evaluations identify a task and attempt to establish a bound on the level of performance that can be elicited from a given model with a given level of investment. The current state of the art is roughly: 1. Choose a reasonable prompting scheme 2. Generate a dataset of high-quality samples and encode them in the chosen format 3. Fine-tune the model and evaluate the resulting performance 4. Make some implicit regularity assumptions about the quality of models fine-tuned using different prompting schemes[1] 5. Conclude that probably no other actor can elicit substantially better performance on the same task from the same model while spending substantially less money than we did This post takes issue with step 4. We begin by illustrating the extreme brittleness of observed model performance when prompting without fine-tuning. Then we argue that fine-tuning is not sufficient to eliminate this effect. Using chess as a toy model, we show two classes of prompting schemes under which ChatGPT-3.5 converges to dramatically different levels of performance after fine-tuning. Our central conclusion is that the structure in which data is presented to an LLM (or at least to ChatGPT 3.5) matters more than one might intuitively expect and that this effect persists through fine-tuning. In the specific case of chess, the better prompting scheme that we use (described in the section below) is easily derived but in situations that are further out of distribution (such as the automated replication and adaptation tasks METR defined), it is not obvious what the best way to present information is, and it seems plausible that there are simple prompt formats which would result in substantially better performance than those that we've tested to date. General Setting We use the term 'agent' to refer to the combination of a model - here gpt-3.5-turbo unless otherwise specified - and a function which takes a chess position as input and outputs the document we feed into the model (henceforth a 'prompting scheme'). We perform our evaluations using three datasets of chess games: 1. A collection of ~6000 games played by humans on Lichess with at least 30 minutes for each player 2. A collection of ~500 games played between all pairings of stockfish 16 level 1, 5, 10, 15, and 20 3. A collection of ~300 games played by ChatGPT 3.5 or gpt-3.5-turbo-instruct with various prompting schemes We evaluate our agents by selecting a random point in each of the games, providing the current game position as...
Duración:00:15:20
EA - Be Proud To Be An Effective Altruist by Omnizoid
6/14/2024
Welcome to The Nonlinear Library, where we use Text-to-Speech software to convert the best writing from the Rationalist and EA communities into audio. This is: Be Proud To Be An Effective Altruist, published by Omnizoid on June 14, 2024 on The Effective Altruism Forum. Crossposted on my blog. When I was at Manifest, Austin Chen said something interesting to me: that one thing that he liked about my blog was that I was proud to be an effective altruist. I didn't treat it as an embarrassing quirk, but as something that's actively good, that's worth encouraging. A lot of EAs don't do this, which I think is sad. Scott Alexander amusingly quips: I hate having to post criticism of EA. Not because EA is bad at taking criticism. The opposite: they like it too much. It almost feels like a sex thing. "Please, tell me again how naughty I'm being!" I went to an EA organization's offices once - I think it was OpenPhil, but don't quote me on that - and the whole place was strewn with the most critical books you can imagine - Robert Reich, Anand Giradharadas, that kind of thing. I remember when I was out tabling for the effective altruism club at my University, some woman came up and started trotting out braindead anti-effective altruism arguments - something about tech bros and SBF and white saviorism. The other very reasonable and responsible EAs at the club were saying things like "hmm, yeah, that's a very interesting criticism, well, if you want to come to a meeting, we'd be happy to discuss it." I wanted to scream! These aren't serious criticisms. This is complete bullshit. Spend enough time listening to the criticisms of effective altruism and it becomes clear that, aside from those arguing for small tweaks at the marigns, they all stem from either a) people being very dogmatic and having a worldview that's strangely incompatible with doing good things (if, for instance, they don't help the communist revolution); b) people wanting an excuse to do nothing in the face of extreme suffering; or c) people disliking effective altruists and so coming up with some half-hearted excuse for why EA is really something-something colonialism. I don't want to be too mean about this, but the criticisms are unbelievably dumb. They are confused to a really extraordinary degree. The reasoning is so exquisitely poor that it's very clearly motivated. I don't say that about other subjects - this is the only subject on which the critics don't have any half-decent objections. And yet despite this, lots of effective altruists treat the criticisms as serious. 1,000 children die a day of malaria. Think about how precious the life is of a young child - concretely picture a small child coughing up blood and lying in bed with a fever of 105. We - the effective altruists - are the ones doing something about that. And not just doing a bit about it - working as hard as possible to eradicate malaria, taking seriously how precious the lives of those children are. GiveWell does research into the charities that avert misery most effectively, and then tens of thousands of EAs all around the world give to those charities, because they recognize that though they'll never see the children they help, those children matter. This is not something to be embarrassed about. Giving money to effective charities and getting others to do the same is by far the best thing I have ever done in my life. Every nice thing I've done interpersonally doesn't have half a percent the value of saving lives, of funneling money into the hands of charities so that little kids don't get horrible diseases that kill them. And yet these critics who do nothing, who sit on their asses as children die whose deaths they can easily avert have the gall to criticize those of us who are doing something, who are working to avert deaths as effectively as possible. They have the gall to criticize those of us who are working to free animals from the horrifying torture chambers known as factory farms. It's one thi...
Duración:00:05:12
LW - AI #68: Remarkably Reasonable Reactions by Zvi
6/14/2024
Welcome to The Nonlinear Library, where we use Text-to-Speech software to convert the best writing from the Rationalist and EA communities into audio. This is: AI #68: Remarkably Reasonable Reactions, published by Zvi on June 14, 2024 on LessWrong. The big news this week was Apple Intelligence being integrated deeply into all their products. Beyond that, we had a modestly better than expected debate over the new version of SB 1047, and the usual tons of stuff in the background. I got to pay down some writing debt. The bad news is, oh no, I have been called for Jury Duty. The first day or two I can catch up on podcasts or pure reading, but after that it will start to hurt. Wish me luck. Table of Contents AiPhone covers the announcement of Apple Intelligence. Apple's products are getting device-wide integration of their own AI in a way they say preserves privacy, with access to ChatGPT via explicit approval for the heaviest requests. A late update: OpenAI is providing this service for free as per Bloomberg. I offered Quotes from Leopold Aschenbrenner's Situational Awareness Paper, attempting to cut down his paper by roughly 80% while still capturing what I considered the key passages. Then I covered his appearance on Dwarkesh's Podcast, where I offered commentary. The plan is to complete that trilogy tomorrow, with a post that analyzes Leopold's positions systematically, and that covers the reactions of others. 1. Introduction. 2. Table of Contents. 3. Language Models Offer Mundane Utility. Roll your own process. 4. Language Models Don't Offer Mundane Utility. What happened to Alexa? 5. Fun With Image Generation. Dude, where's my image of a car? 6. Copyright Confrontation. Everyone is rather on edge these days. 7. Deepfaketown and Botpocalypse Soon. People will do things that scale. 8. They Took Our Jobs. Lost your job? No problem. Start a new company! 9. Someone Explains it All. Data center construction, the bitter lesson. 10. The Art of the Jailbreak. The Most Forbidden Technique? 11. Get Involved. AISI hiring a senior developer. 12. Introducing. New OpenAI execs, new AI assistant, new short video model. 13. In Other AI News. More progress avoiding MatMul. Nvidia takes it all in stride. 14. Quiet Speculations. What you see may be what you get. 15. I Spy With My AI. Microsoft Recall makes some changes to be slightly less crazy. 16. Pick Up the Phone. Perhaps a deal could be made. 17. Lying to the White House, Senate and House of Lords. I don't love it. 18. The Quest for Sane Regulation. People want it. Companies feel differently. 19. More Reasonable SB 1047 Reactions. Hearteningly sane reactions by many. 20. Less Reasonable SB 1047 Reactions. The usual suspects say what you'd suspect. 21. That's Not a Good Idea. Non-AI example, California might ban UV lights. 22. With Friends Like These. Senator Mike Lee has thoughts. 23. The Week in Audio. Lots to choose from, somehow including new Dwarkesh. 24. Rhetorical Innovation. Talking about probabilities with normies is hard. 25. Mistakes Were Made. Rob Bensinger highlights two common ones. 26. The Sacred Timeline. What did you mean? Which ways does it matter? 27. Coordination is Hard. Trying to model exactly how hard it will be. 28. Aligning a Smarter Than Human Intelligence is Difficult. Natural abstractions? 29. People Are Worried About AI Killing Everyone. Reports and theses. 30. Other People Are Not As Worried About AI Killing Everyone. Why not? 31. The Lighter Side. Do you have to do this? What is still in the queue, in current priority order? 1. The third and final post on Leopold Aschenbrenner's thesis will come tomorrow. 2. OpenAI has now had enough drama that I need to cover that. 3. DeepMind's scaling policy will get the analysis it deserves. 4. Other stuff remains: OpenAI model spec, Rand report, Seoul, the Vault. Language Models Offer Mundane Utility Write letters to banks on your behalf by invoking Patrick McKenzie. Can GPT-4 autonomously hack zero-day security flaws u...
Duración:01:18:58
EA - Maybe let the non-EA world train you by ElliotT
6/14/2024
Welcome to The Nonlinear Library, where we use Text-to-Speech software to convert the best writing from the Rationalist and EA communities into audio. This is: Maybe let the non-EA world train you, published by ElliotT on June 14, 2024 on The Effective Altruism Forum. This post is for EAs at the start of their careers who are considering which organisations to apply to, and their next steps in general. Conclusion up front: It can be really hard to get that first job out of university. If you don't get your top picks, your less exciting backup options can still be great for having a highly impactful career. If those first few years of work experience aren't your best pick, they will still be useful as a place where you can 'learn how to job', save some money, and then pivot or grow from there. The main reasons are: The EA job market can be grim. Securing a job at an EA organisation out of university is highly competitive, often resulting in failing to get a job, or chaotic job experiences due to the nascent nature of many EA orgs. An alternative, of getting short-term EA grants to work independently is not much better, as it can lead to financial instability and hinder long-term professional growth. Non-EA jobs have a lot of benefits. They offer a stable and structured environment to build skills, learn organisational norms, get feedback, etc. After, you'll be better placed to do directly impactful work. After a few years at a non-EA job, you'll be better placed to fill a lot of roles at EA orgs. You might also be able to start something yourself. Caveats: Of course, take everything with a grain of salt. For every piece of advice, there is someone who needs to hear the opposite, and the advice in here is no exception. Acknowledgments: Thanks to the following people for giving some great feedback on an a draft of this post and making it better: David Nash, Matt Reardon, Chana Messinger, Karla Still, Michelle Hutchinson. All opinions are my own. All mistakes are chatGPT's. What's the problem? Three failure modes of trying to get an EA job It seems like a lot of people who are motivated by the ideas of effective altruism, use 'get a job at an 'EA org' as shorthand for 'how to have an impact with my career' (this includes me, but more on that later). By EA org I mean the kind of organisation where most people working there are EAs. This is understandable. Figuring out how to have a positive impact with your career is really hard. It's a reasonable heuristic that orgs within the EA community are more likely to have a big positive impact in the world than the average non-profit. Finally, we're all sensitive to status in our community, and in some parts of EA, working at an EA org is definitely considered pretty darn cool. One issue with this that I want to briefly flag is that 'working at an EA org' and 'doing impactful work' are not interchangeable (Michelle at 80,000 Hours covers that well here). But the other thing that you probably know is that EA jobs are really hard to get. They are really, really, competitive. Some jobs get hundreds of high-quality applications. I think this leads to a few failure modes. Story 1 - Lots of rejections: An ambitious, smart, highly engaged EA, fresh out of uni, applies to a lot of EA organisations. They make it far in the hiring rounds, maybe even to trial periods. But, after many disheartening months of applications, trial tasks, and interviews, they don't get an EA job at an EA org. Story 2 - Chaotic EA Job: Alternatively, maybe they get the job at an impactful organisation, thinking they've made it past the hard bit. However, many EA orgs are very young. This can lead to things like a chaotic onboarding, a lack of HR practices, inexperienced managers, and sudden changes in funding or strategy that can leave them without a job. And if that isn't enough, EA branding does not guarantee an org is doing impactful work. Both of these stories are pretty bad. These situations are psychologically and...
Duración:00:11:46
LW - OpenAI appoints Retired U.S. Army General Paul M. Nakasone to Board of Directors by Joel Burget
6/14/2024
Welcome to The Nonlinear Library, where we use Text-to-Speech software to convert the best writing from the Rationalist and EA communities into audio. This is: OpenAI appoints Retired U.S. Army General Paul M. Nakasone to Board of Directors, published by Joel Burget on June 14, 2024 on LessWrong. Today, Retired U.S. Army General Paul M. Nakasone has joined our Board of Directors. A leading expert in cybersecurity, Nakasone's appointment reflects OpenAI's commitment to safety and security, and underscores the growing significance of cybersecurity as the impact of AI technology continues to grow. As a first priority, Nakasone will join the Board's Safety and Security Committee, which is responsible for making recommendations to the full Board on critical safety and security decisions for all OpenAI projects and operations. Whether this was influenced by Aschenbrenner's Situational Awareness or not, it's welcome to see OpenAI emphasizing the importance of security. It's unclear how much this is a gesture vs reflective of deeper changes. Thanks for listening. To help us out with The Nonlinear Library or to learn more, please visit nonlinear.org
Duración:00:01:05
EA - Eight Fundamental Constraints for Growing a Research Organization by Peter Wildeford
6/14/2024
Welcome to The Nonlinear Library, where we use Text-to-Speech software to convert the best writing from the Rationalist and EA communities into audio. This is: Eight Fundamental Constraints for Growing a Research Organization, published by Peter Wildeford on June 14, 2024 on The Effective Altruism Forum. I run a research organization. At any point you want to grow your organization, there will be at least one constraint that keeps it from growing. Over time, I've distilled these into what I consider to be eight fundamental constraints. You may have heard of talent gaps and funding gaps, but I actually think there are eight such gaps. Which constraint it is may change over time, but it is always one of these eight. ~ (1) - There needs to be a sufficient amount of important+neglected+tractable work to support an additional researcher at the research organization. If this constraint is in place, there isn't enough for an additional person to productively do. (People basically never report this as an actual constraint.) ~ (2) - The research organization needs sufficient skill at prioritization, strategy, and vision to ensure they are correctly doing (1) and doing it well. If this constraint is in place, the research organization would not do (1) correctly. (This is commonly referred to as "being bottlenecked on strategic clarity" or "needing more disentanglement".) ~ (3) - The research organization needs a sufficient number of talented researchers available to be hired that could accomplish (1) and (2) if hired. _(This is commonly referred to as a "talent gap".) ~ (4) - There needs to be sufficient people and project management capacity at the research organization to align those hired in (3) with regard to (1) and (2). If this constraint is in place, the research organization cannot direct talented researchers to work on the correct things. ~ (5) - There needs to be sufficient operations capacity to ensure that (1), (2), (3), and (4) all can happen. If this constraint is in place, the research organization may not actually be able to implement hiring rounds or onboard staff, or may risk not being legally compliant. ~ (6) - The research organization needs sufficient funding to pay for all of the above. _(This is commonly referred to as a "funding gap".) ~ (7) - The research organization needs sufficient throughput. That is, even if you have people you want to hire and have the management + operations capacity to have them do good work, it will still take time for people to actually join, get onboarded, become productive, etc. This is an inescapable constraint, but time will keep marching on. ~ (8) - The research organization needs sufficient maintenance of good culture and org happiness so that existing staff feel comfortable with the growth. Thanks for listening. To help us out with The Nonlinear Library or to learn more, please visit nonlinear.org
Duración:00:02:44
AF - Safety isn't safety without a social model (or: dispelling the myth of per se technical safety) by Andrew Critch
6/13/2024
Welcome to The Nonlinear Library, where we use Text-to-Speech software to convert the best writing from the Rationalist and EA communities into audio. This is: Safety isn't safety without a social model (or: dispelling the myth of per se technical safety), published by Andrew Critch on June 14, 2024 on The AI Alignment Forum. As an AI researcher who wants to do technical work that helps humanity, there is a strong drive to find a research area that is definitely helpful somehow, so that you don't have to worry about how your work will be applied, and thus you don't have to worry about things like corporate ethics or geopolitics to make sure your work benefits humanity. Unfortunately, no such field exists. In particular, technical AI alignment is not such a field, and technical AI safety is not such a field. It absolutely matters where ideas land and how they are applied, and when the existence of the entire human race is at stake, that's no exception. If that's obvious to you, this post is mostly just a collection of arguments for something you probably already realize. But if you somehow think technical AI safety or technical AI alignment is somehow intrinsically or inevitably helpful to humanity, this post is an attempt to change your mind. In particular, with more and more AI governance problems cropping up, I'd like to see more and more AI technical staffers forming explicit social models of how their ideas are going to be applied. If you read this post, please don't try to read this post as somehow pro- or contra- a specific area of AI research, or safety, or alignment, or corporations, or governments. My goal in this post is to encourage more nuanced social models by de-conflating a bunch of concepts. This might seem like I'm against the concepts themselves, when really I just want clearer thinking about these concepts, so that we (humanity) can all do a better job of communicating and working together. Myths vs reality Epistemic status: these are claims that I'm confident in, assembled over 1.5 decades of observation of existential risk discourse, through thousands of hours of conversation. They are not claims I'm confident I can convince you of, but I'm giving it a shot anyway because there's a lot at stake when people don't realize how their technical research is going to be misapplied. Myth #1: Technical AI safety and/or alignment advances are intrinsically safe and helpful to humanity, irrespective of the state of humanity. Reality: All technical advances in AI safety and/or "alignment" can be misused by humans. There are no technical advances in AI that are safe per se; the safety or unsafety of an idea is a function of the human environment in which the idea lands. Examples: Obedience - AI that obeys the intention of a human user can be asked to help build unsafe AGI, such as by serving as a coding assistant. (Note: this used to be considered extremely sci-fi, and now it's standard practice.) Interpretability - Tools or techniques for understanding the internals of AI models will help developers better understand what they're building and hence speed up development, possibly exacerbating capabilities races. Truthfulness - AI that is designed to convey true statements to a human can also be asked questions by that human to help them build an unsafe AGI. Myth #2: There's a {technical AI safety VS AI capabilities} dichotomy or spectrum of technical AI research, which also corresponds to {making humanity more safe VS shortening AI timelines}. Reality: Conflating these concepts has three separate problems with it, (a)-(c) below: a) AI safety and alignment advances almost always shorten AI timelines. In particular, the ability to "make an AI system do what you want" is used almost instantly by AI companies to help them ship AI products faster (because the AI does what users want) and to build internal developer tools faster (because the AI does what developers want). (When I point this out, usually people think I'm s...
Duración:00:07:32
EA - Announcing The Midas Project - and our first campaign! by Tyler Johnston
6/13/2024
Welcome to The Nonlinear Library, where we use Text-to-Speech software to convert the best writing from the Rationalist and EA communities into audio. This is: Announcing The Midas Project - and our first campaign!, published by Tyler Johnston on June 13, 2024 on The Effective Altruism Forum. Summary The Midas Project is a new AI safety organization. We use public advocacy to incentivize stronger self-governance from the companies developing and deploying high-risk AI products. This week, we're launching our first major campaign, targeting the AI company Cognition. Cognition is a rapidly growing startup [1] developing autonomous coding agents. Unfortunately, they've told the public virtually nothing about how, or even if, they will conduct risk evaluations to prevent misuse and other unintended outcomes. In fact, they've said virtually nothing about safety at all. We're calling on Cognition to release an industry-standard evaluation-based safety policy. We need your help to make this campaign a success. Here are five ways you can help, sorted by level of effort: 1. Keep in the loop about our campaigns by following us on Twitter and joining our mailing list. 2. Offer feedback and suggestions, by commenting on this post or by reaching out at info@themidasproject.com 3. Share our Cognition campaign on social media, sign the petition, or engage with our campaigns directly on our action hub. 4. Donate to support our future campaigns (tax-exempt status pending). 5. Sign up to volunteer, or express interest in joining our team full-time. Background The risks posed by AI are, at least partially, the result of a market failure. Tech companies are locked in an arms race that is forcing everyone (even the most safety-concerned) to move fast and cut corners. Meanwhile, consumers broadly agree that AI risks are serious and that the industry should move slower. However, this belief is disconnected from their everyday experience with AI products, and there isn't a clear Schelling point allowing consumers to express their preference via the market. Usually, the answer to a market failure like this is regulation. When it comes to AI safety, this is certainly the solution I find most promising. But such regulation isn't happening quickly enough. And even if governments were moving quicker, AI safety as a field is pre-paradigmatic. Nobody knows exactly what guardrails will be most useful, and new innovations are needed. So companies are largely being left to voluntarily implement safety measures. In an ideal world, AI companies would be in a race to the top, competing against each other to earn the trust of the public through comprehensive voluntary safety measures while minimally stifling innovation and the benefits of near-term applications. But the incentives aren't clearly pointing in that direction - at least not yet. However: EA-supported organizations have previously been successful at shifting corporate incentives in the past. Take the case of cage-free campaigns. By engaging in advocacy that threatens to expose specific food companies for falling short of customers' basic expectations regarding animal welfare, groups like The Humane League and Mercy For Animals have been able to create a race to the top for chicken welfare, leading virtually all US food companies to commit to going cage-free. [2] Creating this change was as simple as making the connection in the consumer's mind between their pre-existing disapproval of inhumane battery cages and the eggs being served at their local fast food chain. I believe this sort of public advocacy can be extremely effective. In fact, in the case of previous emerging technologies, I would go so far as to say it's been too effective. Public advocacy played a major role in preventing the widespread adoption of GM crops and nuclear power in the twentieth century, despite huge financial incentives to develop these technologies. [3] We haven't seen this sort of activism leveraged to demand meaningful...
Duración:00:07:45
LW - [Paper] AI Sandbagging: Language Models can Strategically Underperform on Evaluations by Teun van der Weij
6/13/2024
Welcome to The Nonlinear Library, where we use Text-to-Speech software to convert the best writing from the Rationalist and EA communities into audio. This is: [Paper] AI Sandbagging: Language Models can Strategically Underperform on Evaluations, published by Teun van der Weij on June 13, 2024 on LessWrong. We have written a paper on sandbagging for which we present the abstract and brief results in this post. See the paper for more details. Tweet thread here. Abstract Trustworthy capability evaluations are crucial for ensuring the safety of AI systems, and are becoming a key component of AI regulation. However, the developers of an AI system, or the AI system itself, may have incentives for evaluations to understate the AI's actual capability. These conflicting interests lead to the problem of sandbagging - which we define as strategic underperformance on an evaluation. In this paper we assess sandbagging capabilities in contemporary language models (LMs). We prompt frontier LMs, like GPT-4 and Claude 3 Opus, to selectively underperform on dangerous capability evaluations, while maintaining performance on general (harmless) capability evaluations. Moreover, we find that models can be fine-tuned, on a synthetic dataset, to hide specific capabilities unless given a password. This behaviour generalizes to high-quality, held-out benchmarks such as WMDP. In addition, we show that both frontier and smaller models can be prompted, or password-locked, to target specific scores on a capability evaluation. Even more, we found that a capable password-locked model (Llama 3 70b) is reasonably able to emulate a less capable model (Llama 2 7b). Overall, our results suggest that capability evaluations are vulnerable to sandbagging. This vulnerability decreases the trustworthiness of evaluations, and thereby undermines important safety decisions regarding the development and deployment of advanced AI systems. We publish our code at https://github.com/TeunvdWeij/sandbagging Results Evaluators focus on dangerous capability evaluations, where high performance may trigger regulatory interventions. We demonstrate that LMs can be prompted or fine-tuned to selectively underperform on dangerous capability evaluations while maintaining overall performance. Even smaller models can do this using "password-locking", which is fine-tuning models to hide their actual capabilities unless given a password. We show that when Mistral-7b or Llama3-8b are password locked on synthetic data, they still hide capabilities on a high-quality held-out benchmark (WMDP). If a seemingly competent AI system scores surprisingly low on a capability benchmark, detecting sandbagging is easy. However, we find that models can accurately aim for specific scores on benchmarks. Similarly, inconsistent or random performance can be detected. More credibly, strong models could hide capabilities by mimicking weaker models. We demonstrate this capability by successfully fine-tuning Llama 3 70b to emulate Llama 2 7b only when the password is not present. Our work suggests that capability evaluations are vulnerable to sandbagging, which is bad news, but good to know. In our following project, we will work on how to mitigate this problem. Reach out if you are interested in working on this. Thanks for listening. To help us out with The Nonlinear Library or to learn more, please visit nonlinear.org
Duración:00:03:18
EA - Thoughts on the Purpose of EAGs by Tristan Williams
6/13/2024
Welcome to The Nonlinear Library, where we use Text-to-Speech software to convert the best writing from the Rationalist and EA communities into audio. This is: Thoughts on the Purpose of EAGs, published by Tristan Williams on June 13, 2024 on The Effective Altruism Forum. And Other Recommendations for EAGs Based on The Art of The Gathering The Motivation: I read The Art of the Gathering awhile ago, and thought it had a lot of interesting insights for greater community building efforts. I realized that EAGs[1] might be a ripe place to try to apply some of these insights, and so I set out writing this piece. What was meant to be 10 similar-in-length suggestions naturally turned into a piece focused on the purpose of EAGs, but I hope you'll still read over the other points too in the original spirit of the post, I think they have something to offer too. Epistemic Status: All the principles themselves have strong grounding in the book and are things I feel quite confident Parker would endorse. I'll make concrete suggestions below for each, and also venture a long commentary on the potential purpose of EAGs, which are both attempts at applications of these principles and are more speculative and should be taken as "Tristan's best guess". A brief note: CEA and individual EAGx organizers are already doing many of these things to some degree, and as such I hope to not only introduce new ideas or frames, but also highlight and reinforce some of the great things they're already doing. 1. EAGs should have a clear purpose. I think this is the most important point, so I'll spend a great bit of time on it. To put it another way, every gathering needs a greater why that justifies the what. I don't think this is spelled out well for EAGs and has been the cause of some confusion, and I think it would be good to decide on, and then state, a clear why for EAGs. Below I'll mention four different whys I think are at play, and what prioritizing them (more) would mean. For Learning: Talks are consistently an important part of EAGs, and some have argued that they've been underrated[2] in the process of promoting 1-on-1s. Significant staff time is spent organizing the content, giving indication that even if 1-on-1s are considered to be more valuable, there's got to be something worthwhile about them. I think the ToC here is that the talks afford a particularly good opportunity for others to further learn about a topic they're interested in but maybe haven't fully had the chance to explore yet, something likely to be especially valuable for those newer to the EA space. But the talks do actually afford more than this[3]: they are also a sort of podium by which CEA can try to diffuse ideas throughout the community, whether that be promoting a new potential cause area, a better recognition of needs in the EA space, or desirable cultural norms. Focusing the content in 2017 on operations, for example, allowed the community to address a significant gap at the time. They're also a way to expand impact outside the conference when recorded, which could be important as CEA begins to focus more on EA branding and outwards content. Either way, I think many people only have a vague sense of the purpose Learning currently serves, and I think we're even further behind on figuring out how to measure the effectiveness of Learning specifically vs the general impact. Implications: Focus more content on connection. If talks aren't just informational, but also are used to mold the norms of the community both in and outside the conference, maybe more talks should be had in service of helping people make better/more connections. Yes, there's already some of this in the first timers meetup, and motivation in the introductory talks, but I still think far more content should go towards this end given 1-on-1s seems to be the greatest source of value. Be more explicit about the purpose of content at a given EAG. Perhaps there could be some short statement placed in the attende...
Duración:00:40:05
EA - Quantifying and prioritizing shrimp welfare threats by Hannah McKay
6/13/2024
Welcome to The Nonlinear Library, where we use Text-to-Speech software to convert the best writing from the Rationalist and EA communities into audio. This is: Quantifying and prioritizing shrimp welfare threats, published by Hannah McKay on June 13, 2024 on The Effective Altruism Forum. Citation: McKay, H. and McAuliffe, W. (2024). Quantifying and prioritizing shrimp welfare threats. Rethink Priorities. https://doi.org/10.17605/OSF.IO/4QR8K The report is also available on the Rethink Priorities website and as a pdf here . Executive summary This is the fourth report in the Rethink Priorities Shrimp Welfare Sequence. In this report, we quantify the suffering caused to shrimp by 18 welfare threats to assess which welfare issues cause the most harm. See a complete description of the methodology here. We focused on penaeid shrimp in ongrowing farms and broodstock facilities. Incorporating uncertainty at each step of the model, we estimated the prevalence, intensity, and duration of pain caused by each welfare issue. The intensity was based on the Welfare Footprint Project's Pain-Track categories, and 'pain' refers to their definition of pain, encapsulating both physical and mental negative experiences ( Alonso & Schuck-Paim, 2024a). We collapse different pain type estimates into a single metric: 'Disabling-equivalent pain'. See the results in Figure 1. The average farmed shrimp spends 154 hours in disabling-equivalent pain (95% Subjective Credible Interval (SCI): [13, 378]). If we assume that 608 billion penaeid shrimp die on ongrowing farms annually (i.e., including those that die pre-slaughter; Waldhorn & Autric, 2023) then mean values imply that they experience 94 trillion hours of disabling-equivalent pain a year (95% SCI: [8 trillion, 230 trillion]). The highest-ranking threats are chronic issues that affect most farmed shrimp. The top three are high stocking density, high un-ionized ammonia, and low dissolved oxygen. Threats ranked lower are broadly acute, one-off events affecting only a subpopulation (e.g., eyestalk ablation, which affects only broodstock). However, the credible intervals are too wide to determine the rank order of most welfare issues confidently. Box 1: Shrimp aquaculture terminology The terms 'shrimp' and 'prawn' are often used interchangeably. The two terms do not reliably track any phylogenetic differences between species. Here, we use only the term "shrimp", covering both shrimp and prawns. Note that members of the family Artemiidae are commonly referred to as "brine shrimp" but are not decapods and so are beyond the present scope. We opt for the use of Penaues vannamei over Litopenaeus vannamei (to which this species is often referred), due to recognition of the former but not the latter nomenclature by ITIS, WorMS, and the Food and Agriculture Organization of the United Nations (FAO) ASFIS List of Species for Fishery Statistics Purposes. The shrimp farming industry uses many terms usually associated with agriculture - for example, 'crops' for a group of shrimp reared together, 'seed' for the first shrimp stocked into a pond, and 'harvest' for collecting and slaughtering shrimp. For clarity, we broadly conform to this terminology. Although we acknowledge animal welfare advocates may prefer terminology that does not euphemize or sanitize the experience of farmed shrimp, here we favor ensuring readability for a wide audience. Introduction We began the Shrimp Welfare Sequence by asking whether the Animal Sentience Precautionary Principle ( Birch, 2017, p. 3) justifies implementing reforms in shrimp aquaculture. The first three posts collectively provide an affirmative answer: More shrimp are alive on farms than any other farmed taxa Half of them die before slaughter, suggesting that some of the welfare threats they endure must be serious. The welfare threats shrimp experience are varied, ranging from poor water quality to environmental deprivation to inhumane slaughter. Unfortunately, it is probably n...
Duración:00:27:51