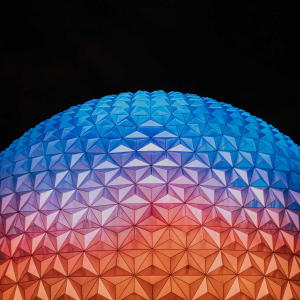
Vector Podcast
Technology Podcasts
Vector Podcast is here to bring you the depth and breadth of Search Engine Technology, Product, Marketing, Business. In the podcast we talk with engineers, entrepreneurs, thinkers and tinkerers, who put their soul into search. Depending on your interest, you should find a matching topic for you -- whether it is deep algorithmic aspect of search engines and information retrieval field, or examples of products offering deep tech to its users. "Vector" -- because it aims to cover an emerging field of vector similarity search, giving you the ability to search content beyond text: audio, video, images and more. "Vector" also because it is all about vector in your profession, product, marketing and business.
Location:
Finland
Genres:
Technology Podcasts
Description:
Vector Podcast is here to bring you the depth and breadth of Search Engine Technology, Product, Marketing, Business. In the podcast we talk with engineers, entrepreneurs, thinkers and tinkerers, who put their soul into search. Depending on your interest, you should find a matching topic for you -- whether it is deep algorithmic aspect of search engines and information retrieval field, or examples of products offering deep tech to its users. "Vector" -- because it aims to cover an emerging field of vector similarity search, giving you the ability to search content beyond text: audio, video, images and more. "Vector" also because it is all about vector in your profession, product, marketing and business.
Twitter:
@DmitryKan
Language:
English
Debunking myths of vector search and LLMs with Leo Boytsov
Duration:01:07:54
Berlin Buzzwords 2024 - Alessandro Benedetti - LLMs in Solr
Duration:00:38:04
Berlin Buzzwords 2024 - Sonam Pankaj - EmbedAnything
Duration:00:23:00
Berlin Buzzwords 2024 - Doug Turnbull - Learning in Public
Duration:00:27:29
Eric Pugh - Measuring Search Quality with Quepid
Duration:00:47:37
Sid Probstein, part II - Bring AI to company data with SWIRL
Duration:00:38:15
Louis Brandy - SQL meets Vector Search at Rockset
Duration:00:52:50
Saurabh Rai - Growing Resume Matcher
Duration:00:26:15
Sid Probstein - Creator of SWIRL - Search in siloed data with LLMs
Duration:01:32:23
Atita Arora - Search Relevance Consultant - Revolutionizing E-commerce with Vector Search
Duration:01:32:20
Connor Shorten - Research Scientist, Weaviate - ChatGPT, LLMs, Form vs Meaning
Duration:01:33:11
Evgeniya Sukhodolskaya - Data Advocate, Toloka - Data at the core of all the cool ML
Duration:01:26:45
Yaniv Vaknin - Director of Product, Searchium - Hardware accelerated vector search
Duration:01:13:24
Doug Turnbull - Staff Relevance Engineer, Shopify - Search as a constant experimentation cycle
Duration:01:24:55
Malte Pietsch - CTO, Deepset - Passion in NLP and bridging the academia-industry gap with Haystack
Duration:01:25:59
Max Irwin - Founder, MAX.IO - On economics of scale in embedding computation with Mighty
Duration:01:51:27
Grant Ingersoll - Fractional CTO, Leading Search Consultant - Engineering Better Search
Duration:01:12:39
Daniel Tunkelang - Leading Search Consultant - Leveraging ML for query and content understanding
Duration:01:02:28
Yusuf Sarıgöz - AI Research Engineer, Qdrant - Getting to know your data with metric learning
Duration:01:02:12
Jo Bergum - Distinguished Engineer, Yahoo! Vespa - Journey of Vespa from Sparse into Neural Search
Duration:00:30:51