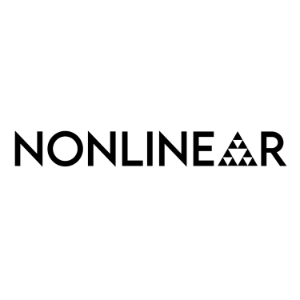
The Nonlinear Library
Education Podcasts
The Nonlinear Library allows you to easily listen to top EA and rationalist content on your podcast player. We use text-to-speech software to create an automatically updating repository of audio content from the EA Forum, Alignment Forum, LessWrong, and other EA blogs. To find out more, please visit us at nonlinear.org
Location:
United States
Genres:
Education Podcasts
Description:
The Nonlinear Library allows you to easily listen to top EA and rationalist content on your podcast player. We use text-to-speech software to create an automatically updating repository of audio content from the EA Forum, Alignment Forum, LessWrong, and other EA blogs. To find out more, please visit us at nonlinear.org
Language:
English
Episodes
EA - Announcing the EA Nigeria Summit by EA Nigeria
5/23/2024
Welcome to The Nonlinear Library, where we use Text-to-Speech software to convert the best writing from the Rationalist and EA communities into audio. This is: Announcing the EA Nigeria Summit, published by EA Nigeria on May 23, 2024 on The Effective Altruism Forum. We are excited to announce the EA Nigeria Summit, which will take place on September 6th and 7th, 2024, in Abuja, Nigeria. The two-night event aims to bring together individuals thinking carefully about some of the world's biggest problems, taking impactful action to solve them, or exploring ways to do so. Attendees can share knowledge, network, and explore collaboration opportunities with like-minded people and organizations from Nigeria, Africa, and other international attendees. We are organizing the summit with the support of the Centre for Effective Altruism Event Team. The summit is open to individuals from Nigeria, Africa, or other international locations, and we expect to welcome 100+ individuals at the summit; emphasis will be given to the following categories of individuals: Existing members of the EA Nigeria community or Nigerian individuals familiar with effective altruism. African Individuals who are familiar with and engaging with the EA community Individuals (International or local) running or working for EA-aligned projects with operations in Nigeria or other African states. International individuals who could contribute to the event's sessions. Unfortunately, we have limited capacity for the summit, so we will have to choose who we accept based on who we think would get the most out of it. However, these are not exhaustive, and we encourage you to apply even if you are in doubt! We can also provide invitation letters for a visitor visa for the summit for international individuals but can't provide additional help and can't guarantee that this letter will be sufficient. Learn more about the summit and apply here, application is open until August 5th, 2024. For inquiries or questions, feel free to comment under this post or email us at info@eanigeria.org. We hope to see you there! Thanks for listening. To help us out with The Nonlinear Library or to learn more, please visit nonlinear.org
Duration:00:02:02
AF - Paper in Science: Managing extreme AI risks amid rapid progress by JanB
5/23/2024
Welcome to The Nonlinear Library, where we use Text-to-Speech software to convert the best writing from the Rationalist and EA communities into audio. This is: Paper in Science: Managing extreme AI risks amid rapid progress, published by JanB on May 23, 2024 on The AI Alignment Forum. https://www.science.org/doi/10.1126/science.adn0117 Authors: Yoshua Bengio, Geoffrey Hinton, Andrew Yao, Dawn Song, Pieter Abbeel, Yuval Noah Harari, Ya-Qin Zhang, Lan Xue, Shai Shalev-Shwartz, Gillian Hadfield, Jeff Clune, Tegan Maharaj, Frank Hutter, Atılım Güneş Baydin, Sheila McIlraith, Qiqi Gao, Ashwin Acharya, David Krueger, Anca Dragan, Philip Torr, Stuart Russell, Daniel Kahneman, Jan Brauner*, Sören Mindermann* Abstract: Artificial intelligence (AI) is progressing rapidly, and companies are shifting their focus to developing generalist AI systems that can autonomously act and pursue goals. Increases in capabilities and autonomy may soon massively amplify AI's impact, with risks that include large-scale social harms, malicious uses, and an irreversible loss of human control over autonomous AI systems. Although researchers have warned of extreme risks from AI, there is a lack of consensus about how to manage them. Society's response, despite promising first steps, is incommensurate with the possibility of rapid, transformative progress that is expected by many experts. AI safety research is lagging. Present governance initiatives lack the mechanisms and institutions to prevent misuse and recklessness and barely address autonomous systems. Drawing on lessons learned from other safety-critical technologies, we outline a comprehensive plan that combines technical research and development with proactive, adaptive governance mechanisms for a more commensurate preparation. Thanks for listening. To help us out with The Nonlinear Library or to learn more, please visit nonlinear.org.
Duration:00:01:58
LW - "Which chains-of-thought was that faster than?" by Emrik
5/23/2024
Welcome to The Nonlinear Library, where we use Text-to-Speech software to convert the best writing from the Rationalist and EA communities into audio. This is: "Which chains-of-thought was that faster than?", published by Emrik on May 23, 2024 on LessWrong. Here's some good advice from Eliezer: TAP: "How could I have thought that faster?" WHEN[1] you complete a chain-of-thought THEN ask yourself, "how could I have thought that faster?" I really like this heuristic, and it's already paid its rent several times over for me. Most recently today, so I'll share the (slightly edited) cognitive trace of it as an example: Example: To find the inverse of something, trace the chain forward a few times first 1. I was in the context of having just asked myself "what's the set of functions which have this function as its derivative?" 2. This is of course its integral, but I didn't want to use cached abstractions, and instead sought to get a generalized view of the landscape from first-principles. 3. For about ~10 seconds, I tried to hold the function f in my mind while trying to directly generate the integral landscape from it. 4. This seemed awfwly inefficient, so I changed tack: I already know some specific functions whose derivatives equal f, so I held those as the proximal thing in my mind while retracing the cognitive steps involved in their derivation. 5. After making those steps more salient in the forward direction (integralderivative), it was easier to retrace the path in the opposite direction. 6. And once the derivativeintegral trace was salient for a few examples, it was easier to generalize from the examples to produce the landscape of all the integrals. 7. There are multiple takeaways here, but one is: 1. "If you struggle to generalize something, find a way to generate specific examples first, then generalize from the examples." TAP: "Which chains-of-thought was that faster than?" Imo, more important than asking "how could I have thought that faster?" is the inverse heuristic: WHEN you complete a good chain-of-thought THEN ask yourself, "which chains-of-thought was that faster than?" Although, ideally, I wouldn't scope the trigger to every time you complete a thought, since that overburdens the general cue. Instead, maybe limit it to those times when you have an especially clear trace of it AND you have a hunch that something about it was unusually good. WHEN you complete a good chain of thought AND you have its trace in short-term memory AND you hunch that something about it was unusually effective THEN ask yourself, "which chains-of-thought was that faster than?" Example: Sketching out my thoughts with pen-and-paper 1. Yesterday I was writing out some plans explicitly with pen and paper - enumerating my variables and drawing arrows between them. 2. I noticed - for the umpteenth time - that forcing myself to explicitly sketch out the problem (even with improvised visualizations) is far more cognitively ergonomic than keeping it in my head (see eg why you should write pseudocode). 3. But instead of just noting "yup, I should force myself to do more pen-and-paper", I asked myself two questions: 1. "When does it help me think, and when does it just slow me down?" 1. This part is important: scope your insight sharply to contexts where it's usefwl - hook your idea into the contexts where you want it triggered - so you avoid wasting memory-capacity on linking it up to useless stuff. 2. In other words, you want to minimize (unwanted) associative interference so you can remember stuff at lower cost. 3. My conclusion was that pen-and-paper is good when I'm trying to map complex relations between a handfwl of variables. 4. And it is NOT good when I have just a single proximal idea that I want to compare against a myriad of samples with high false-positive rate - that's instead where I should be doing inside-head thinking to exploit the brain's massively parallel distributed processor. 2. "Why am I so reluctant to do it?" 1. This se...
Duration:00:07:40
EA - Survey: bioethicists' views on bioethical issues by Leah Pierson
5/22/2024
Welcome to The Nonlinear Library, where we use Text-to-Speech software to convert the best writing from the Rationalist and EA communities into audio. This is: Survey: bioethicists' views on bioethical issues, published by Leah Pierson on May 22, 2024 on The Effective Altruism Forum. Summary Bioethicists influence practices and policies in medicine, science, and public health. However, little is known about bioethicists' views in aggregate. We recently surveyed 824 U.S bioethicists on a wide range of ethical issues, including several issues of interest to the EA community (e.g., compensating organ donors, priority setting, paternalistic regulations, and trade-offs between human and animal welfare, among others). We aimed to contact everyone who presented at the American Society for Bioethics and Humanities Annual Conference in 2021 or 2022 and/or is affiliated with a US bioethics training program. Of the 1,713 people contacted, 824 (48%) completed the survey. Why should EAs care? 1. As Devin Kalish puts it in this nice post: "Bioethics is the field of ethics that focuses on issues like pandemics, human enhancement, AI, global health, animal rights, and environmental ethics. Bioethicists, in short, have basically the same exact interests as us." 2. Many EAs don't hold the bioethics community in high regard. Much of this animus seems to stem from EAs' perception that bioethicists have bad takes. (See Devin's post for more on this.) Our survey casts light on bioethicists' views; people can update their opinions accordingly. What did we find? Chris Said of Apollo Surveys[1] separately analyzed our data and wrote a blog post summarizing our results: Primary results A large majority (87%) of bioethicists believed that abortion was ethically permissible. 82% thought it was permissible to select embryos based on somewhat painful medical conditions, whereas only 22% thought it was permissible to select on non-medical traits like eye color or height. 59% thought it was ethically permissible for clinicians to assist patients in ending their own lives. 15% of bioethicists thought it was ethically permissible to offer payment in exchange for organs (e.g. kidneys). Question 1 Please provide your opinion on whether the following actions are ethically permissible. Is abortion ethically permissible? Is it ethically permissible to select some embryos over others for gestation on the basis of somewhat painful medical conditions? Is it ethically permissible to make trade-offs between human welfare and non-human animal welfare? Is it ethically permissible for a clinician to treat a 14-year-old for opioid use disorder without their parents' knowledge or consent? Is it ethically permissible to offer payment in exchange for blood products? Is it ethically permissible to subject people to regulation they disagree with, solely for the sake of their own good? Is it ethically permissible for clinicians to assist patients in ending their own lives if they request this? Is it ethically permissible for a government to allow an individual to access treatments that have not been approved by regulatory agencies, but only risk harming that individual and not others? Is it ethically permissible to consider an individual's past decisions when determining their access to medical resources? Is it ethically permissible to select some embryos over others for gestation on the basis of non-medical traits (e.g., eye color, height)? Is it ethically permissible to offer payment in exchange for organs (e.g., kidneys)? Is it ethically permissible for decisional surrogates to make a medical decision that they believe is in a patient's best interest, even when that decision goes against the patient's previously stated preferences? Is it ethically permissible for a clinician to provide life-saving care to an adult patient who has refused that care and has decision-making capacity? Results Question 2 In general, should policymakers consider non-health benefits and harms (lik...
Duration:00:05:36
LW - Do Not Mess With Scarlett Johansson by Zvi
5/22/2024
Welcome to The Nonlinear Library, where we use Text-to-Speech software to convert the best writing from the Rationalist and EA communities into audio. This is: Do Not Mess With Scarlett Johansson, published by Zvi on May 22, 2024 on LessWrong. I repeat. Do not mess with Scarlett Johansson. You would think her movies, and her suit against Disney, would make this obvious. Apparently not so. Andrej Karpathy (co-founder OpenAI, departed earlier), May 14: The killer app of LLMs is Scarlett Johansson. You all thought it was math or something. You see, there was this voice they created for GPT-4o, called 'Sky.' People noticed it sounded suspiciously like Scarlett Johansson, who voiced the AI in the movie Her, which Sam Altman says is his favorite movie of all time, which he says inspired OpenAI 'more than a little bit,' and then he tweeted "Her" on its own right before the GPT-4o presentation, and which was the comparison point for many people reviewing the GPT-4o debut? Quite the Coincidence I mean, surely that couldn't have been intentional. Oh, no. Kylie Robison: I asked Mira Mutari about Scarlett Johansson-type voice in today's demo of GPT-4o. She clarified it's not designed to mimic her, and said someone in the audience asked this exact same question! Kylie Robison in Verge (May 13): Title: ChatGPT will be able to talk to you like Scarlett Johansson in Her. OpenAI reports on how it created and selected its five selected GPT-4o voices. OpenAI: We support the creative community and worked closely with the voice acting industry to ensure we took the right steps to cast ChatGPT's voices. Each actor receives compensation above top-of-market rates, and this will continue for as long as their voices are used in our products. We believe that AI voices should not deliberately mimic a celebrity's distinctive voice - Sky's voice is not an imitation of Scarlett Johansson but belongs to a different professional actress using her own natural speaking voice. To protect their privacy, we cannot share the names of our voice talents. … Looking ahead, you can expect even more options as we plan to introduce additional voices in ChatGPT to better match the diverse interests and preferences of users. Jessica Taylor: My "Sky's voice is not an imitation of Scarlett Johansson" T-shirt has people asking a lot of questions already answered by my shirt. OpenAI: We've heard questions about how we chose the voices in ChatGPT, especially Sky. We are working to pause the use of Sky while we address them. Variety: Altman said in an interview last year that "Her" is his favorite movie. Variety: OpenAI Suspends ChatGPT Voice That Sounds Like Scarlett Johansson in 'Her': AI 'Should Not Deliberately Mimic a Celebrity's Distinctive Voice.' [WSJ had similar duplicative coverage.] Flowers from the Future: That's why we can't have nice things. People bore me. Again: Do not mess with Scarlett Johansson. She is Black Widow. She sued Disney. Several hours after compiling the above, I was happy to report that they did indeed mess with Scarlett Johansson. She is pissed. Bobby Allen (NPR): Scarlett Johansson says she is 'shocked, angered' over new ChatGPT voice. … Johansson's legal team has sent OpenAI two letters asking the company to detail the process by which it developed a voice the tech company dubbed "Sky," Johansson's publicist told NPR in a revelation that has not been previously reported. NPR then published her statement, which follows. Scarlett Johansson's Statement Scarlett Johansson: Last September, I received an offer from Sam Altman, who wanted to hire me to voice the current ChatGPT 4.0 system. He told me that he felt that by my voicing the system, I could bridge the gap between tech companies and creatives and help consumers to feel comfortable with the seismic shift concerning humans and Al. He said he felt that my voice would be comforting to people. After much consideration and for personal reasons, I declined the offer. Nine months later, my friends,...
Duration:00:25:24
EA - Summary: Against the singularity hypothesis by Global Priorities Institute
5/22/2024
Welcome to The Nonlinear Library, where we use Text-to-Speech software to convert the best writing from the Rationalist and EA communities into audio. This is: Summary: Against the singularity hypothesis, published by Global Priorities Institute on May 22, 2024 on The Effective Altruism Forum. This is a summary of the GPI Working Paper "Against the singularity hypothesis" by David Thorstad (published in Philosophical Studies). The summary was written by Riley Harris. The singularity is a hypothetical future event in which machines rapidly become significantly smarter than humans. The idea is that we might invent an artificial intelligence (AI) system that can improve itself. After a single round of self-improvement, that system would be better equipped to improve itself than before. This process might repeat many times, and each time the AI system would become more capable and better equipped to improve itself even further. At the end of this (perhaps very rapid) process, the AI system could be much smarter than the average human. Philosophers and computer scientists have thought we should take the possibility of a singularity seriously (Solomonoff 1985, Good 1996, Chalmers 2010, Bostrom 2014, Russell 2019). It is characteristic of the singularity hypothesis that AI will take years or months at the most to become many times more intelligent than even the most intelligent human.[1] Such extraordinary claims require extraordinary evidence. In the paper "Against the singularity hypothesis", David Thorstad claims that we do not have enough evidence to justify the belief in the singularity hypothesis, and we should consider it unlikely unless stronger evidence emerges. Reasons to think the singularity is unlikely Thorstad is sceptical that machine intelligence can grow quickly enough to justify the singularity hypothesis. He gives several reasons for this. Low-hanging fruit. Innovative ideas and technological improvements tend to become more difficult over time. For example, consider "Moore's law", which is (roughly) the observation that hardware capacities double every two years. Between 1971 and 2014 Moore's law was maintained only with an astronomical increase in the amount of capital and labour invested into semiconductor research (Bloom et al. 2020). In fact, according to one leading estimate, there was an eighteen-fold drop in productivity over this period. While some features of future AI systems will allow them to increase the rate of progress compared to human scientists and engineers, they are still likely to experience diminishing returns as the easiest discoveries have already been made and only more difficult ideas are left. Bottlenecks. AI progress relies on improvements in search, computation, storage and so on (each of these areas breaks down into many subcomponents). Progress could be slowed down by any of these subcomponents: if any of these are difficult to speed up, then AI progress will be much slower than we would naively expect. The classic metaphor here concerns the speed a liquid can exit a bottle, which is rate-limited by the narrow space near the opening. AI systems may run into bottlenecks if any essential components cannot be improved quickly (see Aghion et al., 2019). Constraints. Resource and physical constraints may also limit the rate of progress. To take an analogy, Moore's law gets more difficult to maintain because it is expensive, physically difficult and energy-intensive to cram ever more transistors in the same space. Here we might expect progress to eventually slow as physical and financial constraints provide ever greater barriers to maintaining progress. Sublinear growth. How do improvements in hardware translate to intelligence growth? Thompson and colleagues (2022) find that exponential hardware improvements translate to linear gains in performance on problems such as Chess, Go, protein folding, weather prediction and the modelling of underground oil reservoirs. Over the past 50 years,...
Duration:00:09:22
EA - Scorable Functions: A Format for Algorithmic Forecasting by Ozzie Gooen
5/22/2024
Welcome to The Nonlinear Library, where we use Text-to-Speech software to convert the best writing from the Rationalist and EA communities into audio. This is: Scorable Functions: A Format for Algorithmic Forecasting, published by Ozzie Gooen on May 22, 2024 on The Effective Altruism Forum. Introduction Imagine if a forecasting platform had estimates for things like: 1. "For every year until 2100, what will be the probability of a global catastrophic biological event, given different levels of biosecurity investment and technological advancement?" 2. "What will be the impact of various AI governance policies on the likelihood of developing safe and beneficial artificial general intelligence, and how will this affect key indicators of global well-being over the next century?" 3. "How valuable is every single project funded by Open Philanthropy, according to a person with any set of demographic information, if they would spend 1000 hours reflecting on it?" These complex, multidimensional questions are useful for informing decision-making and resource allocation around effective altruism and existential risk mitigation. However, traditional judgemental forecasting methods often struggle to capture the nuance and conditionality required to address such questions effectively. This is where "scorable functions" come in - a forecasting format that allows forecasters to directly submit entire predictive models rather than just point estimates or simple probability distributions. Scorable functions allow encoding a vast range of relationships and dependencies, from basic linear trends to intricate nonlinear dynamics. Forecasters can precisely specify interactions between variables, the evolution of probabilities over time, and how different scenarios could unfold. At their core, scorable functions are executable models that output probabilistic predictions and can be directly scored via function calls. They encapsulate the forecasting logic, whether it stems from human judgment, data-driven insights, or a hybrid of the two. Scorable functions can span from concise one-liners to elaborate constructs like neural networks. Over the past few years, we at QURI have been investigating how to effectively harness these methods. We believe scorable functions could be a key piece of the forecasting puzzle going forward. From Forecast Bots to Scorable Functions Many people are familiar with the idea of using "bots" to automate forecasts on platforms like Metaculus. Let's consider a simple example to see how scorable functions can extend this concept. Suppose there's a binary question on Metaculus: "Will event X happen in 2024?" Intuitively, the probability should decrease as 2024 progresses, assuming no resolution. A forecaster might start at 90% in January, but want to gradually decrease to 10% by December. One approach is to manually update the forecast each week - a tedious process. A more efficient solution is to write a bot that submits forecasts based on a simple function: (Example using Squiggle, but hopefully it's straightforward enough) This bot can automatically submit daily forecasts via the Metaculus API. However, while more efficient than manual updates, this approach has several drawbacks: 1. The platform must store and process a separate forecast for each day, even though they all derive from a simple function. 2. Viewers can't see the full forecast trajectory, only the discrete submissions. 3. The forecaster's future projections and scenario contingencies are opaque. Scorable functions elegantly solve these issues. Instead of a bot submitting individual forecasts, the forecaster simply submits the generating function itself. You can imagine there being a custom input box directly in Metaculus. The function submitted would be the same, though it might be provided as a lambda function or with a standardized function name. The platform can then evaluate this function on-demand to generate up-to-date forecasts. Viewers see the comp...
Duration:00:17:01
LW - Anthropic announces interpretability advances. How much does this advance alignment? by Seth Herd
5/22/2024
Welcome to The Nonlinear Library, where we use Text-to-Speech software to convert the best writing from the Rationalist and EA communities into audio. This is: Anthropic announces interpretability advances. How much does this advance alignment?, published by Seth Herd on May 22, 2024 on LessWrong. Anthropic just published a pretty impressive set of results in interpretability. This raises for me, some questions and a concern: Interpretability helps, but it isn't alignment, right? It seems to me as though the vast bulk of alignment funding is now going to interpretability. Who is thinking about how to leverage interpretability into alignment? It intuitively seems as though we are better off the more we understand the cognition of foundation models. I think this is true, but there are sharp limits: it will be impossible to track the full cognition of an AGI, and simply knowing what it's thinking about will be inadequate to know whether it's making plans you like. One can think about bioweapons, for instance, to either produce them or prevent producing them. More on these at the end; first a brief summary of their results. In this work, they located interpretable features in Claude 3 Sonnet using sparse autoencoders, and manipulating model behavior using those features as steering vectors. They find features for subtle concepts; they highlight features for: The Golden Gate Bridge 34M/31164353: Descriptions of or references to the Golden Gate Bridge. Brain sciences 34M/9493533: discussions of neuroscience and related academic research on brains or minds. Monuments and popular tourist attractions 1M/887839. Transit infrastructure 1M/3. [links to examples] ... We also find more abstract features - responding to things like bugs in computer code, discussions of gender bias in professions, and conversations about keeping secrets. ...we found features corresponding to: Capabilities with misuse potential (code backdoors, developing biological weapons) Different forms of bias (gender discrimination, racist claims about crime) Potentially problematic AI behaviors (power-seeking, manipulation, secrecy) Presumably, the existence of such features will surprise nobody who's used and thought about large language models. It is difficult to imagine how they would do what they do without using representations of subtle and abstract concepts. They used the dictionary learning approach, and found distributed representations of features: Our general approach to understanding Claude 3 Sonnet is based on the linear representation hypothesis and the superposition hypothesis from the publication, Scaling Monosemanticity: Extracting Interpretable Features from Claude 3 Sonnet. Or to put it more plainly: It turns out that each concept is represented across many neurons, and each neuron is involved in representing many concepts. Representations in the brain definitely follow that description, and the structure of representations seems pretty similar as far as we can guess from animal studies and limited data on human language use. They also include a fascinating image of near neighbors to the feature for internal conflict (see header image). So, back to the broader question: it is clear how this type of interpretability helps with AI safety: being able to monitor when it's activating features for things like bioweapons, and use those features as steering vectors, can help control the model's behavior. It is not clear to me how this generalizes to AGI. And I am concerned that too few of us are thinking about this. It seems pretty apparent how detecting lying will dramatically help in pretty much any conceivable plan for technical alignment of AGI. But it seems like being able to monitor an entire thought process of a being smarter than us is impossible on the face of it. I think the hope is that we can detect and monitor cognition that is about dangerous topics, so we don't need to follow its full train of thought. If we can tell what an AGI is thinking ...
Duration:00:06:06
AF - Announcing Human-aligned AI Summer School by Jan Kulveit
5/22/2024
Welcome to The Nonlinear Library, where we use Text-to-Speech software to convert the best writing from the Rationalist and EA communities into audio. This is: Announcing Human-aligned AI Summer School, published by Jan Kulveit on May 22, 2024 on The AI Alignment Forum. The fourth Human-aligned AI Summer School will be held in Prague from 17th to 20th July 2024. We will meet for four intensive days of talks, workshops, and discussions covering latest trends in AI alignment research and broader framings of AI alignment research. Apply now , applications are evaluated on a rolling basis. The intended audience of the school are people interested in learning more about the AI alignment topics, PhD students, researchers working in ML/AI outside academia, and talented students. Format of the school The school is focused on teaching and exploring approaches and frameworks, less on presentation of the latest research results. The content of the school is mostly technical - it is assumed the attendees understand current ML approaches and some of the underlying theoretical frameworks. This year, the school will cover these main topics: Overview of the alignment problem and current approaches. Alignment of large language models: RLHF, DPO and beyond. Methods used to align current large language models and their shortcomings. Evaluating and measuring AI systems: How to understand and oversee current AI systems on the behavioral level. Interpretability and the science of deep learning: What's going on inside of the models? AI alignment theory: While 'prosaic' approaches to alignment focus on current systems, theory aims for deeper understanding and better generalizability. Alignment in the context of complex systems and multi-agent settings: What should the AI be aligned to? In most realistic settings, we can expect there are multiple stakeholders and many interacting AI systems; any solutions to alignment problem need to solve multi-agent settings. The school consists of lectures and topical series, focused smaller-group workshops and discussions, expert panels, and opportunities for networking, project brainstorming and informal discussions. Detailed program of the school will be announced shortly before the event. See below for a program outline and e.g. the program of the previous school for an illustration of the program content and structure. Confirmed speakers Stephen Casper - Algorithmic Alignment Group, MIT. Stanislav Fort - Google DeepMind. Jesse Hoogland - Timaeus. Jan Kulveit - Alignment of Complex Systems, Charles University. Mary Phuong - Google DeepMind. Deger Turan - AI Objectives Institute and Metaculus. Vikrant Varma - Google DeepMind. Neel Nanda - Google DeepMind. (more to be announced later) Thanks for listening. To help us out with The Nonlinear Library or to learn more, please visit nonlinear.org.
Duration:00:02:47
EA - The Charity Commission has concluded its inquiry into Effective Ventures Foundation UK by Rob Gledhill
5/22/2024
Welcome to The Nonlinear Library, where we use Text-to-Speech software to convert the best writing from the Rationalist and EA communities into audio. This is: The Charity Commission has concluded its inquiry into Effective Ventures Foundation UK, published by Rob Gledhill on May 22, 2024 on The Effective Altruism Forum. The Charity Commission for England and Wales has concluded its statutory inquiry into Effective Ventures Foundation UK (EVF UK), which was originally launched in 2023 following the collapse of FTX. The full report on the inquiry can be found here, and the Commission's press release on the inquiry can be found here. The inquiry's scope was to examine: The extent of any risk to EVF's assets. The extent to which the trustees were complying with their legal obligations to protect the charity's property The governance and administration of the charity by the trustees.[1] We are pleased that "the inquiry found that the trustees took appropriate steps to protect the charity's funds and complied with their legal duties acting diligently and quickly following the collapse of FTX." The Commission's report notes the full cooperation of EVF's trustees and that they "sought to act in the charity's best interests." Although the Commission noted that there had been a "lack of clarity" around historical conflicts of interest and a lack of formal process for identifying conflicts of interest, "in practice no issues arose" and "there is no evidence to suggest that there were any unmanaged conflicts of interest regarding funds the charity received from the FTX Foundation or that any trustee had acted in a way contrary to the interests of the charity." They also note that subsequent to FTX's collapse, "Both the finance and legal teams at the charity have been strengthened and policies have been bolstered or created with more robust frameworks." I'm pleased that the charity commission recognises the improvements that have been made at EV. This report doesn't change EV's strategy to decentralise, as previously announced here. 1. ^ For further context, the Charity Commission is a regulator in the UK whose responsibilities include: preventing mismanagement and misconduct by charities; promoting compliance with charity law; protecting the property, beneficiaries, and work of charities; and safeguarding the public's trust and confidence in charities. A statutory inquiry is a tool for the Commission to establish facts and collect evidence related to these responsibilities. Thanks for listening. To help us out with The Nonlinear Library or to learn more, please visit nonlinear.org
Duration:00:02:18
EA - A tale of two Sams by Geoffrey Miller
5/22/2024
Welcome to The Nonlinear Library, where we use Text-to-Speech software to convert the best writing from the Rationalist and EA communities into audio. This is: A tale of two Sams, published by Geoffrey Miller on May 22, 2024 on The Effective Altruism Forum. After Sam Bankman-Fried proved to be a sociopathic fraudster and a massive embarrassment to EA, we did much soul-searching about what EAs did wrong, in failing to detect and denounce his sociopathic traits. We spent, collectively, thousands of hours ruminating about what we can do better, next time we encounter an unprincipled leader who acts like it's OK to abuse and betray people to pursue their grandiose vision, who gets caught up in runaway greed for wealth and power, who violates core EA values, and who threatens the long-term flourishing of sentient beings. Well, that time is now. Sam Altman at OpenAI has been proving himself, again and again, in many different domains and issues, to be a manipulative, deceptive, unwise, and arrogant leader, driven by hubris to build AGI as fast as possible, with no serious concern about the extinction risks he's imposing on us all. We are all familiar with the recent controversies and scandals at OpenAI, from the boardroom coup, to the mass violations of intellectual property in training LLMs, to the collapse of the Superalignment Team, to the draconian Non-Disparagement Agreements, to the new Scarlett Johansson voice emulation scandal this week. The evidence for Sam Altman being a Bad Actor seems, IMHO, at least as compelling as the evidence for Sam Bankman-Fried being a Bad Actor before the FTX collapse in Nov 2022. And the stakes are much, much higher for humanity (if not for EA's reputation). So what are we going to do about it? Should we keep encouraging young talented EAs to go work in the AI industry, in the hopes that they can nudge the AI companies from the inside towards safe AGI alignment -- despite the fact that many of them end up quitting, disillusioned and frustrated? Should we keep making excuses for OpenAI, and Anthropic, and DeepMind, pursuing AGI at recklessly high speed, despite the fact that AI capabilities research is far out-pacing AI safety and alignment research? Should we keep offering the public the hope that 'AI alignment' is a solvable problem, when we have no evidence that aligning AGIs with 'human values' would be any easier than aligning Palestinians with Israeli values, or aligning libertarian atheists with Russian Orthodox values -- or even aligning Gen Z with Gen X values? I don't know. But if we feel any culpability or embarrassment about the SBF/FTX debacle, I think we should do some hard thinking about how to deal with the OpenAI debacle. Many of us work on AI safety, and are concerned about extinction risks. I worry that all of our efforts in these directions could be derailed by a failure to call out the second rich, influential, pseudo-EA, sociopathic Sam that we've learned about in the last two years. If OpenAI 'succeeds' in developing AGI within a few years, long before we have any idea how to control AGI, that could be game over for our species. Especially if Sam Altman and his supporters and sycophants are still running OpenAI. [Epistemic note: I've written this hastily, bluntly, with emotion, because I think there's some urgency to EA addressing these issues.] Thanks for listening. To help us out with The Nonlinear Library or to learn more, please visit nonlinear.org
Duration:00:03:08
EA - The suffering of a farmed animal is equal in size to the happiness of a human, according to a survey by Stijn
5/21/2024
Welcome to The Nonlinear Library, where we use Text-to-Speech software to convert the best writing from the Rationalist and EA communities into audio. This is: The suffering of a farmed animal is equal in size to the happiness of a human, according to a survey, published by Stijn on May 21, 2024 on The Effective Altruism Forum. Author: Stijn Bruers, researcher economics KU Leuven Short summary According to a survey among a representative sample of the Belgian population, most people believe that farmed animals like chickens have the same capacity for suffering as humans, and that most farmed land animals (broiler chickens) have negative welfare levels (i.e. experience more suffering than happiness). The average suffering of a farmed land animal, estimated by people, is equal in size to the positive welfare of an average human (in Belgium) whereas the welfare level of a wild bird is zero on average. Given the fact that there are more farmed animals than humans in the world, and that the populations of small farmed animals (chickens, fish, shrimp and insects) are increasing, most people would have to come to the conclusion that net global welfare (of humans, farmed animals and wild animals combined) is negative and declining. People who care about global welfare should therefore strongly prioritize decreasing animal farming and improving farmed animal welfare conditions. Introduction How much do farmed animals such as broiler chickens suffer? How can we compare the welfare of animals and humans? These are crucially important questions, because knowing the welfare capacities and welfare levels of humans and non-human animals is necessary to prioritize strategies to improve welfare on Earth. They can also be used to estimate the global welfare state of the world, as was first done by Fish (2023). His results were very pessimistic: net global welfare may be negative and declining, due to the increased farming of small animals (chicken, fish, shrimp and possibly insects). The top-priority to improve global welfare and decrease suffering on Earth becomes very clear: decrease animal farming (or decrease the suffering of farmed animals). Fish arrived at these pessimistic results using welfare range and welfare level estimates by animal welfare experts at Rethink Priorities (the Moral Weight Project) and Charity Entrepreneurship (the Weighted Animal Welfare Index). However, the calculations by Fish may be criticized on the point that his choice of welfare ranges and welfare levels was too arbitrary, because it first involved the arbitrary choice of source or group of experts, and those experts themselves also made arbitrary choices to arrive at their welfare range and level estimates. Perhaps people believe that the welfare capacities and levels of animal suffering used by Fish were overestimated? Perhaps people won't believe his results because they don't believe that animals have such high capacities for suffering? In order to convince the general public, we can instead consider the estimates of welfare ranges and welfare levels of animals given by the wider public. To do so, a survey among a representative sample of the Flemish population in Belgium was conducted to study how much sentience people ascribe to non-human animals. The estimates of animal welfare ranges by the general public were more animal-positive than those of Rethink Priorities. Most respondents gave higher values of animal welfare ranges than those given by the animal welfare experts at Rethink Priorities. According to the general public, Rethink Priorities may have underestimated the animal welfare ranges. Furthermore, most people estimate that the welfare level of most farmed land animals (chickens) is negative, and in absolute value as large as the positive welfare level of humans (in line with the Animal Welfare Index estimates by Charity Entrepreneurship). Hence, according to the general public, the results of Fish were too optimistic. The global welfare sta...
Duration:00:26:24
AF - EIS XIII: Reflections on Anthropic's SAE Research Circa May 2024 by Stephen Casper
5/21/2024
Welcome to The Nonlinear Library, where we use Text-to-Speech software to convert the best writing from the Rationalist and EA communities into audio. This is: EIS XIII: Reflections on Anthropic's SAE Research Circa May 2024, published by Stephen Casper on May 21, 2024 on The AI Alignment Forum. Part 13 of 12 in the Engineer's Interpretability Sequence. TL;DR On May 5, 2024, I made a set of 10 predictions about what the next sparse autoencoder (SAE) paper from Anthropic would and wouldn't do. Today's new SAE paper from Anthropic was full of brilliant experiments and interesting insights, but it ultimately underperformed my expectations. I am beginning to be concerned that Anthropic's recent approach to interpretability research might be better explained by safety washing than practical safety work. Think of this post as a curt editorial instead of a technical piece. I hope to revisit my predictions and this post in light of future updates. Reflecting on predictions Please see my original post for 10 specific predictions about what today's paper would and wouldn't accomplish. I think that Anthropic obviously did 1 and 2 and obviously did not do 4, 5, 7, 8, 9, and 10. Meanwhile, I think that their experiments to identify specific and safety-relevant features should count for 3 (proofs of concept for a useful type of task) but definitely do not count for 6 (*competitively* finding and removing a harmful behavior that was represented in the training data). Thus, my assessment is that Anthropic did 1-3 but not 4-10. I have been wrong with mech interp predictions in the past, but this time, I think I was 10 for 10: everything I predicted with >50% probability happened, and everything I predicted with <50% probability did not happen. Overall, the paper underperformed my expectations. If you scored the paper relative to my predictions by giving it (1-p) points when it did something that I predicted it would do with probability p and -p points when it did not, the paper would score -0.74. A review + thoughts I think that Anthropic's new SAE work has continued to be like lots of prior high-profile work on mechanistic interpretability - it has focused on presenting illustrative examples, streetlight demos, and cherry-picked proofs of concept. This is useful for science, but it does not yet show that SAEs are helpful and competitive for diagnostic and debugging tasks that could improve AI safety. I feel increasingly concerned about how Anthropic motivates and sells its interpretability research in the name of safety. Today's paper makes some major Motte and Bailey claims that oversell what was accomplished like " Eight months ago, we demonstrated that sparse autoencoders could recover monosemantic features from a small one-layer transformer," " Sparse autoencoders produce interpretable features for large models," and " The resulting features are highly abstract: multilingual, multimodal, and generalizing between concrete and abstract references." The paper also made some omissions of past literature on interpretability illusions (e.g., Bolukbasi et al., 2021), which their methodology seems prone to. Normally, problems like this are mitigated by peer review, which Anthropic does not participate in. Meanwhile, whenever Anthropic puts out new interpretability research, I see a laundry list of posts from the company and employees to promote it. They always seem to claim the same thing - that some 'groundbreaking new progress has been made' and that 'the model was even more interpretable than they thought' but that 'there remains progress to be made before interpretability is solved'. I won't link to any specific person's posts, but here is Anthropic's post from today and October 2023. The way that Anthropic presents its interpretability work has real-world consequences. For example, it seems to have led to viral claims that interpretability will be solved and that we are bound for safe models. It has also led to at least one claim in a pol...
Duration:00:05:50
LW - On Dwarkesh's Podcast with OpenAI's John Schulman by Zvi
5/21/2024
Welcome to The Nonlinear Library, where we use Text-to-Speech software to convert the best writing from the Rationalist and EA communities into audio. This is: On Dwarkesh's Podcast with OpenAI's John Schulman, published by Zvi on May 21, 2024 on LessWrong. Dwarkesh Patel recorded a Podcast with John Schulman, cofounder of OpenAI and at the time their head of current model post-training. Transcript here. John's job at the time was to make the current AIs do what OpenAI wanted them to do. That is an important task, but one that employs techniques that their at-the-time head of alignment, Jan Leike, made clear we should not expect to work on future more capable systems. I strongly agree with Leike on that. Then Sutskever left and Leike resigned, and John Schulman was made the new head of alignment, now charged with what superalignment efforts remain at OpenAI to give us the ability to control future AGIs and ASIs. This gives us a golden opportunity to assess where his head is at, without him knowing he was about to step into that role. There is no question that John Schulman is a heavyweight. He executes and ships. He knows machine learning. He knows post-training and mundane alignment. The question is, does he think well about this new job that has been thrust upon him? The Big Take Overall I was pleasantly surprised and impressed. In particular, I was impressed by John's willingness to accept uncertainty and not knowing things. He does not have a good plan for alignment, but he is far less confused about this fact than most others in similar positions. He does not know how to best navigate the situation if AGI suddenly happened ahead of schedule in multiple places within a short time frame, but I have not ever heard a good plan for that scenario, and his speculations seem about as directionally correct and helpful as one could hope for there. Are there answers that are cause for concern, and places where he needs to fix misconceptions as quickly as possible? Oh, hell yes. His reactions to potential scenarios involved radically insufficient amounts of slowing down, halting and catching fire, freaking out and general understanding of the stakes. Some of that I think was about John and others at OpenAI using a very weak definition of AGI (perhaps partly because of the Microsoft deal?) but also partly he does not seem to appreciate what it would mean to have an AI doing his job, which he says he expects in a median of five years. His answer on instrumental convergence is worrisome, as others have pointed out. He dismisses concerns that an AI given a bounded task would start doing things outside the intuitive task scope, or the dangers of an AI 'doing a bunch of wacky things' a human would not have expected. On the plus side, it shows understanding of the key concepts on a basic (but not yet deep) level, and he readily admits it is an issue with commands that are likely to be given in practice, such as 'make money.' In general, he seems willing to react to advanced capabilities by essentially scaling up various messy solutions in ways that I predict would stop working at that scale or with something that outsmarts you and that has unanticipated affordances and reason to route around typical in-distribution behaviors. He does not seem to have given sufficient thought to what happens when a lot of his assumptions start breaking all at once, exactly because the AI is now capable enough to be properly dangerous. As with the rest of OpenAI, another load-bearing assumption is presuming gradual changes throughout all this, including assuming past techniques will not break. I worry that will not hold. He has some common confusions about regulatory options and where we have viable intervention points within competitive dynamics and game theory, but that's understandable, and also was at the time very much not his department. As with many others, there seems to be a disconnect. A lot of the thinking here seems like excellent practical thi...
Duration:00:33:25
LW - New voluntary commitments (AI Seoul Summit) by Zach Stein-Perlman
5/21/2024
Welcome to The Nonlinear Library, where we use Text-to-Speech software to convert the best writing from the Rationalist and EA communities into audio. This is: New voluntary commitments (AI Seoul Summit), published by Zach Stein-Perlman on May 21, 2024 on LessWrong. Basically the companies commit to make responsible scaling policies. Part of me says this is amazing, the best possible commitment short of all committing to a specific RSP. It's certainly more real than almost all other possible kinds of commitments. But as far as I can tell, people pay almost no attention to what RSP-ish documents (Anthropic, OpenAI, Google) actually say and whether the companies are following them. The discourse is more like "Anthropic, OpenAI, and Google have safety plans and other companies don't." Hopefully that will change. Maybe "These commitments represent a crucial and historic step forward for international AI governance." It does seem nice from an international-governance perspective that Mistral AI, TII, and a Chinese company joined. The UK and Republic of Korea governments announced that the following organisations have agreed to the Frontier AI Safety Commitments: Amazon Anthropic Cohere Google G42 IBM Inflection AI Meta Microsoft Mistral AI Naver OpenAI Samsung Electronics Technology Innovation Institute xAI Zhipu.ai The above organisations, in furtherance of safe and trustworthy AI, undertake to develop and deploy their frontier AI models and systems[1] responsibly, in accordance with the following voluntary commitments, and to demonstrate how they have achieved this by publishing a safety framework focused on severe risks by the upcoming AI Summit in France. Given the evolving state of the science in this area, the undersigned organisations' approaches (as detailed in paragraphs I-VIII) to meeting Outcomes 1, 2 and 3 may evolve in the future. In such instances, organisations will provide transparency on this, including their reasons, through public updates. The above organisations also affirm their commitment to implement current best practices related to frontier AI safety, including: internal and external red-teaming of frontier AI models and systems for severe and novel threats; to work toward information sharing; to invest in cybersecurity and insider threat safeguards to protect proprietary and unreleased model weights; to incentivize third-party discovery and reporting of issues and vulnerabilities; to develop and deploy mechanisms that enable users to understand if audio or visual content is AI-generated; to publicly report model or system capabilities, limitations, and domains of appropriate and inappropriate use; to prioritize research on societal risks posed by frontier AI models and systems; and to develop and deploy frontier AI models and systems to help address the world's greatest challenges. Outcome 1. Organisations effectively identify, assess and manage risks when developing and deploying their frontier AI models and systems. They will: I. Assess the risks posed by their frontier models or systems across the AI lifecycle, including before deploying that model or system, and, as appropriate, before and during training. Risk assessments should consider model capabilities and the context in which they are developed and deployed, as well as the efficacy of implemented mitigations to reduce the risks associated with their foreseeable use and misuse. They should also consider results from internal and external evaluations as appropriate, such as by independent third-party evaluators, their home governments[2], and other bodies their governments deem appropriate. II. Set out thresholds[3] at which severe risks posed by a model or system, unless adequately mitigated, would be deemed intolerable. Assess whether these thresholds have been breached, including monitoring how close a model or system is to such a breach. These thresholds should be defined with input from trusted actors, including organisations' respective ho...
Duration:00:12:00
EA - Introducing MSI Reproductive Choices by Meghan Blake
5/21/2024
Welcome to The Nonlinear Library, where we use Text-to-Speech software to convert the best writing from the Rationalist and EA communities into audio. This is: Introducing MSI Reproductive Choices, published by Meghan Blake on May 21, 2024 on The Effective Altruism Forum. In 1976, our founder Tim Black established MSI Reproductive Choices[1] to bring contraception and abortion care to women in underserved communities that no one else would go to. As a doctor, he witnessed firsthand the hardship caused by the lack of reproductive choice and accordingly, he established quality care, cost-effectiveness, data, and sustainability as foundational principles of the organization. Nearly 50 years later, this legacy remains at the core of MSI Reproductive Choices today. Since our founding, MSI has served more than 200 million clients, of which more than 100 million were served in the last nine years. Since 2000, our global services have averted an estimated 316,000 maternal deaths and 158.6 million unintended pregnancies. We deliver impact with exceptional cost-effectiveness. On a global scale via our Outreach and Public Sector Strengthening programs, which reach underserved "last mile" communities, our average cost per disability-adjusted life year (DALY) is $4.70[2], and our average cost per maternal death averted is $3,353. In Nigeria, our most cost-effective program, these figures drop to just $1.63 per DALY and $685 per maternal death averted in our programming with the most underserved communities.[3] The Challenge Health Benefits: Family planning saves lives and is a development intervention that brings transformational benefits to women, their families, and communities. While progress has been made, it hasn't been fast enough; 257 million people still lack access to contraception, resulting in 111 million unintended pregnancies annually. Additionally, 280,000 women - primarily in sub-Saharan Africa - lose their lives due to pregnancy-related complications each year, amounting to 767 deaths per day. Maternal mortality is nearly 50 times higher for women in sub-Saharan Africa compared to high-income countries, and their babies are 10 times more likely to die in their first month of life. The global disparity between the rate of maternal deaths is evident in low-income countries. In 2020, the Maternal Mortality Ratio (MMR) reached 430 per 100,000 live births in low-income countries, a significant contrast to just 12 per 100,000 live births in high-income nations. In some countries, like Nigeria, the maternal mortality rate exceeds 1,000 per 100,000 live births. Demand for family planning and sexual and reproductive healthcare services will continue to grow, and by 2030, an additional 180 million women will need access to these services. The urgency of this need is emphasized by the adolescent girls in low- and middle-income countries who wish to avoid pregnancy, yet a significant 43% of them face an unmet need for contraception. Pregnancy-related deaths are the leading cause of death for adolescent girls globally. The World Health Organization has stressed that to avoid maternal deaths, it is vital to prevent unintended pregnancies. They stated: "all women, including adolescents, need access to contraception, safe abortion services to the full extent of the law, and quality post-abortion care." If all women in low- and middle-income countries who wish to avoid pregnancy had access to family planning, the rate of unintended pregnancies would drop by 68%. Number of Maternal Deaths by Region, 2000 - 2017 Education and Economic Opportunities: The effects of inadequate access to family planning are profoundly felt in Sub-Saharan Africa, where every year, MSI analysis has estimated that up to 4 million teenage girls drop out of school due to teenage pregnancy. This education gap is exacerbated by disparities in contraceptive access: women in the wealthiest quintile have more than double the proportion of met contraceptive demand compa...
Duration:00:19:31
LW - [Linkpost] Statement from Scarlett Johansson on OpenAI's use of the "Sky" voice, that was shockingly similar to her own voice. by Linch
5/21/2024
Welcome to The Nonlinear Library, where we use Text-to-Speech software to convert the best writing from the Rationalist and EA communities into audio. This is: [Linkpost] Statement from Scarlett Johansson on OpenAI's use of the "Sky" voice, that was shockingly similar to her own voice., published by Linch on May 21, 2024 on LessWrong. Scarlett Johansson makes a statement about the "Sky" voice, a voice for GPT-4o that OpenAI recently pulled after less than a week of prime time. tl;dr: OpenAI made an offer last September to Johansson; she refused. They offered again 2 days before the public demo. Scarlett Johansson claims that the voice was so similar that even friend and family noticed. She hired legal counsel to ask OpenAI to "detail the exact process by which they created the 'Sky' voice," which resulted in OpenAI taking the voice down. Full statement below: Last September, I received an offer from Sam Altman, who wanted to hire me to voice the current ChatGPT 4.0 system. He told me that he felt that by my voicing the system, I could bridge the gap between tech companies and creatives and help consumers to feel comfortable with the seismic shift concerning humans and Al. He said he felt that my voice would be comforting to people. After much consideration and for personal reasons, declined the offer. Nine months later, my friends, family and the general public all noted how much the newest system named 'Sky' sounded like me. When I heard the released demo, I was shocked, angered and in disbelief that Mr. Altman would pursue a voice that sounded so eerily similar to mine that my closest friends and news outlets could not tell the difference. Mr. Altman even insinuated that the similarity was intentional, tweeting a single word 'her' - a reference to the film in which I voiced a chat system, Samantha, who forms an intimate relationship with a human. Two days before the ChatGPT 4.0 demo was released, Mr. Altman contacted my agent, asking me to reconsider. Before we could connect, the system was out there. As a result of their actions, I was forced to hire legal counsel, who wrote two letters to Mr. Altman and OpenAl, setting out what they had done and asking them to detail the exact process by which they created the 'Sky' voice. Consequently, OpenAl reluctantly agreed to take down the 'Sky' voice. In a time when we are all grappling with deepfakes and the protection of our own likeness, our own work, our own identities, I believe these are questions that deserve absolute clarity. I look forward to resolution in the form of transparency and the passage of appropriate legislation to help ensure that individual rights are protected. Thanks for listening. To help us out with The Nonlinear Library or to learn more, please visit nonlinear.org
Duration:00:02:31
AF - The Problem With the Word 'Alignment' by peligrietzer
5/20/2024
Welcome to The Nonlinear Library, where we use Text-to-Speech software to convert the best writing from the Rationalist and EA communities into audio. This is: The Problem With the Word 'Alignment', published by peligrietzer on May 21, 2024 on The AI Alignment Forum. This post was written by Peli Grietzer, inspired by internal writings by TJ (tushant jha), for AOI[1]. The original post, published on Feb 5, 2024, can be found here: https://ai.objectives.institute/blog/the-problem-with-alignment. The purpose of our work at the AI Objectives Institute (AOI) is to direct the impact of AI towards human autonomy and human flourishing. In the course of articulating our mission and positioning ourselves -- a young organization -- in the landscape of AI risk orgs, we've come to notice what we think are serious conceptual problems with the prevalent vocabulary of 'AI alignment.' This essay will discuss some of the major ways in which we think the concept of 'alignment' creates bias and confusion, as well as our own search for clarifying concepts. At AOI, we try to think about AI within the context of humanity's contemporary institutional structures: How do contemporary market and non-market (eg. bureaucratic, political, ideological, reputational) forces shape AI R&D and deployment, and how will the rise of AI-empowered corporate, state, and NGO actors reshape those forces? We increasingly feel that 'alignment' talk tends to obscure or distort these questions. The trouble, we believe, is the idea that there is a single so-called Alignment Problem. Talk about an 'Alignment Problem' tends to conflate a family of related but distinct technical and social problems, including: P1: Avoiding takeover from emergent optimization in AI agents P2: Ensuring that AI's information processing (and/or reasoning) is intelligible to us P3: Ensuring AIs are good at solving problems as specified (by user or designer) P4: Ensuring AI systems enhance, and don't erode, human agency P5: Ensuring that advanced AI agents learn a human utility function P6: Ensuring that AI systems lead to desirable systemic and long term outcomes Each of P1-P6 is known as 'the Alignment Problem' (or as the core research problem in 'Alignment Research') to at least some people in the greater AI Risk sphere, in at least some contexts. And yet these problems are clearly not simply interchangeable: placing any one of P1-P6 at the center of AI safety implies a complicated background theory about their relationship, their relative difficulty, and their relative significance. We believe that when different individuals and organizations speak of the 'Alignment Problem,' they assume different controversial reductions of the P1-P6 problems network to one of its elements. Furthermore, the very idea of an 'Alignment Problem' precommits us to finding a reduction for P1-P6, obscuring the possibility that this network of problems calls for a multi-pronged treatment. One surface-level consequence of the semantic compression around 'alignment' is widespread miscommunication, as well as fights over linguistic real-estate. The deeper problem, though, is that this compression serves to obscure some of a researcher's or org's foundational ideas about AI by 'burying' them under the concept of alignment. Take a familiar example of a culture clash within the greater AI Risk sphere: many mainstream AI researchers identify 'alignment work' with incremental progress on P3 (task-reliability), which researchers in the core AI Risk community reject as just safety-washed capabilities research. We believe working through this culture-clash requires that both parties state their theories about the relationship between progress on P3 and progress on P1 (takeover avoidance). In our own work at AOI, we've had occasion to closely examine a viewpoint we call the Berkeley Model of Alignment -- a popular reduction of P1-P6 to P5 (agent value-learning) based on a paradigm consolidated at UC Berkeley's CHAI research gr...
Duration:00:10:54
EA - What's Going on With OpenAI's Messaging? by Ozzie Gooen
5/20/2024
Welcome to The Nonlinear Library, where we use Text-to-Speech software to convert the best writing from the Rationalist and EA communities into audio. This is: What's Going on With OpenAI's Messaging?, published by Ozzie Gooen on May 21, 2024 on The Effective Altruism Forum. This is a quickly-written opinion piece, of what I understand about OpenAI. I first posted it to Facebook, where it had some discussion. Some arguments that OpenAI is making, simultaneously: 1. OpenAI will likely reach and own transformative AI (useful for attracting talent to work there). 2. OpenAI cares a lot about safety (good for public PR and government regulations). 3. OpenAI isn't making anything dangerous and is unlikely to do so in the future (good for public PR and government regulations). 4. OpenAI doesn't need to spend many resources on safety, and implementing safe AI won't put it at any competitive disadvantage (important for investors who own most of the company). 5. Transformative AI will be incredibly valuable for all of humanity in the long term (for public PR and developers). 6. People at OpenAI have thought long and hard about what will happen, and it will be fine. 7. We can't predict concretely what transformative AI will look like or what will happen after (Note: Any specific scenario they propose would upset a lot of people. Vague hand-waving upsets fewer people). 8. OpenAI can be held accountable to the public because it has a capable board of advisors overseeing Sam Altman (he said this explicitly in an interview). 9. The previous board scuffle was a one-time random event that was a very minor deal. 10. OpenAI has a nonprofit structure that provides an unusual focus on public welfare. 11. The nonprofit structure of OpenAI won't inconvenience its business prospects or shareholders in any way. 12. The name "OpenAI," which clearly comes from the early days when the mission was actually to make open-source AI, is an equally good name for where the company is now.* (I don't actually care about this, but find it telling that the company doubles down on arguing the name still is applicable). So they need to simultaneously say: "We're making something that will dominate the global economy and outperform humans at all capabilities, including military capabilities, but is not a threat." "Our experimental work is highly safe, but in a way that won't actually cost us anything." "We're sure that the long-term future of transformative change will be beneficial, even though none of us can know or outline specific details of what that might actually look like." "We have a great board of advisors that provide accountability. Sure, a few months ago, the board tried to fire Sam, and Sam was able to overpower them within two weeks, but next time will be different." "We have all of the benefits of being a nonprofit, but we don't have any of the costs of being a nonprofit." Meta's messaging is clearer. "AI development won't get us to transformative AI, we don't think that AI safety will make a difference, we're just going to optimize for profitability." Anthropic's messaging is a bit clearer "We think that AI development is a huge deal and correspondingly scary, and we're taking a costlier approach accordingly, though not too costly such that we'd be irrelevant." This still requires a strange and narrow worldview to make sense, but it's still more coherent. But OpenAI's messaging has turned into a particularly tangled mess of conflicting promises. It's the kind of political strategy that can work for a while, especially if you can have most of your conversations in private, but is really hard to pull off when you're highly public and facing multiple strong competitive pressures. If I were a journalist interviewing Sam Altman, I'd try to spend as much of it as possible just pinning him down on these countervailing promises they're making. Some types of questions I'd like him to answer would include: "Please lay out a specific, year-by-year, story of o...
Duration:00:05:49
LW - Anthropic: Reflections on our Responsible Scaling Policy by Zac Hatfield-Dodds
5/20/2024
Welcome to The Nonlinear Library, where we use Text-to-Speech software to convert the best writing from the Rationalist and EA communities into audio. This is: Anthropic: Reflections on our Responsible Scaling Policy, published by Zac Hatfield-Dodds on May 20, 2024 on LessWrong. Last September we published our first Responsible Scaling Policy (RSP) [LW discussion], which focuses on addressing catastrophic safety failures and misuse of frontier models. In adopting this policy, our primary goal is to help turn high-level safety concepts into practical guidelines for fast-moving technical organizations and demonstrate their viability as possible standards. As we operationalize the policy, we expect to learn a great deal and plan to share our findings. This post shares reflections from implementing the policy so far. We are also working on an updated RSP and will share this soon. We have found having a clearly-articulated policy on catastrophic risks extremely valuable. It has provided a structured framework to clarify our organizational priorities and frame discussions around project timelines, headcount, threat models, and tradeoffs. The process of implementing the policy has also surfaced a range of important questions, projects, and dependencies that might otherwise have taken longer to identify or gone undiscussed. Balancing the desire for strong commitments with the reality that we are still seeking the right answers is challenging. In some cases, the original policy is ambiguous and needs clarification. In cases where there are open research questions or uncertainties, setting overly-specific requirements is unlikely to stand the test of time. That said, as industry actors face increasing commercial pressures we hope to move from voluntary commitments to established best practices and then well-crafted regulations. As we continue to iterate on and improve the original policy, we are actively exploring ways to incorporate practices from existing risk management and operational safety domains. While none of these domains alone will be perfectly analogous, we expect to find valuable insights from nuclear security, biosecurity, systems safety, autonomous vehicles, aerospace, and cybersecurity. We are building an interdisciplinary team to help us integrate the most relevant and valuable practices from each. Our current framework for doing so is summarized below, as a set of five high-level commitments. 1. Establishing Red Line Capabilities. We commit to identifying and publishing "Red Line Capabilities" which might emerge in future generations of models and would present too much risk if stored or deployed under our current safety and security practices (referred to as the ASL-2 Standard). 2. Testing for Red Line Capabilities (Frontier Risk Evaluations). We commit to demonstrating that the Red Line Capabilities are not present in models, or - if we cannot do so - taking action as if they are (more below). This involves collaborating with domain experts to design a range of "Frontier Risk Evaluations" - empirical tests which, if failed, would give strong evidence against a model being at or near a red line capability. We also commit to maintaining a clear evaluation process and a summary of our current evaluations publicly. 3. Responding to Red Line Capabilities. We commit to develop and implement a new standard for safety and security sufficient to handle models that have the Red Line Capabilities. This set of measures is referred to as the ASL-3 Standard. We commit not only to define the risk mitigations comprising this standard, but also detail and follow an assurance process to validate the standard's effectiveness. Finally, we commit to pause training or deployment if necessary to ensure that models with Red Line Capabilities are only trained, stored and deployed when we are able to apply the ASL-3 standard. 4. Iteratively extending this policy. Before we proceed with activities which require the ASL-3 standard, we commit...
Duration:00:17:21