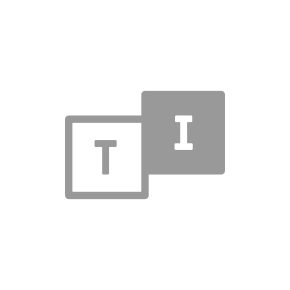
Latent Space: The AI Engineer Podcast — Practitioners talking LLMs, CodeGen, Agents, Multimodality, AI UX, GPU Infra and all things Software 3.0
Technology Podcasts
The podcast by and for AI Engineers! In 2023, over 1 million visitors came to Latent Space to hear about news, papers and interviews in Software 3.0. We cover Foundation Models changing every domain in Code Generation, Multimodality, AI Agents, GPU Infra and more, directly from the founders, builders, and thinkers involved in pushing the cutting edge. Striving to give you both the definitive take on the Current Thing down to the first introduction to the tech you'll be using in the next 3 months! We break news and exclusive interviews from OpenAI, tiny (George Hotz), Databricks/MosaicML (Jon Frankle), Modular (Chris Lattner), Answer.ai (Jeremy Howard), et al. Full show notes always on https://latent.space
www.latent.space
Location:
United States
Description:
The podcast by and for AI Engineers! In 2023, over 1 million visitors came to Latent Space to hear about news, papers and interviews in Software 3.0. We cover Foundation Models changing every domain in Code Generation, Multimodality, AI Agents, GPU Infra and more, directly from the founders, builders, and thinkers involved in pushing the cutting edge. Striving to give you both the definitive take on the Current Thing down to the first introduction to the tech you'll be using in the next 3 months! We break news and exclusive interviews from OpenAI, tiny (George Hotz), Databricks/MosaicML (Jon Frankle), Modular (Chris Lattner), Answer.ai (Jeremy Howard), et al. Full show notes always on https://latent.space www.latent.space
Language:
English
Website:
https://www.latent.space/podcast
WebSim, WorldSim, and The Summer of Simulative AI — with Joscha Bach of Liquid AI, Karan Malhotra of Nous Research, Rob Haisfield of WebSim.ai
Duration:00:53:47
High Agency Pydantic > VC Backed Frameworks — with Jason Liu of Instructor
Duration:00:52:20
Supervise the Process of AI Research — with Jungwon Byun and Andreas Stuhlmüller of Elicit
Duration:00:56:20
Latent Space Chats: NLW (Four Wars, GPT5), Josh Albrecht/Ali Rohde (TNAI), Dylan Patel/Semianalysis (Groq), Milind Naphade (Nvidia GTC), Personal AI (ft. Harrison Chase — LangFriend/LangMem)
Duration:02:02:20
Presenting the AI Engineer World's Fair — with Sam Schillace, Deputy CTO of Microsoft
Duration:00:42:58
Why Google failed to make GPT-3 + why Multimodal Agents are the path to AGI — with David Luan of Adept
Duration:00:41:52
Making Transformers Sing - with Mikey Shulman of Suno
Duration:00:52:51
Top 5 Research Trends + OpenAI Sora, Google Gemini, Groq Math (Jan-Feb 2024 Audio Recap) + Latent Space Anniversary with Lindy.ai, RWKV, Pixee, Julius.ai, Listener Q&A!
Duration:01:48:52
Open Source AI is AI we can Trust — with Soumith Chintala of Meta AI
Duration:01:20:00
A Brief History of the Open Source AI Hacker - with Ben Firshman of Replicate
Duration:01:10:04
Truly Serverless Infra for AI Engineers - with Erik Bernhardsson of Modal
Duration:01:02:25
Cloud Intelligence at the speed of 5000 tok/s - with Ce Zhang and Vipul Ved Prakash of Together AI
Duration:01:03:11
Why StackOverflow usage is down 50% — with David Hsu of Retool
Duration:00:57:20
The Four Wars of the AI Stack (Dec 2023 Audio Recap)
Duration:01:08:18
How to train your own Large Multimodal Model — with Hugo Laurençon & Leo Tronchon of HuggingFace M4
Duration:01:11:50
RLHF 201 - with Nathan Lambert of AI2 and Interconnects
Duration:01:25:30
The Accidental AI Canvas - with Steve Ruiz of tldraw
Duration:01:04:09
NeurIPS 2023 Recap — Top Startups
Duration:02:41:54
NeurIPS 2023 Recap — Best Papers
Duration:03:20:26
The AI-First Graphics Editor - with Suhail Doshi of Playground AI
Duration:00:57:09