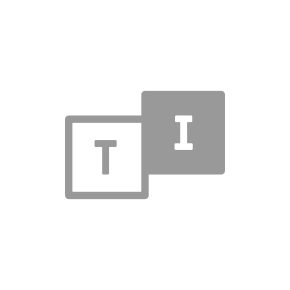
Papers Read on AI
Education Podcasts
Keeping you up to date with the latest trends and best performing architectures in this fast evolving field in computer science. Selecting papers by comparative results, citations and influence we educate you on the latest research. Consider supporting us on Patreon.com/PapersRead for feedback and ideas.
Location:
Iceland
Genres:
Education Podcasts
Description:
Keeping you up to date with the latest trends and best performing architectures in this fast evolving field in computer science. Selecting papers by comparative results, citations and influence we educate you on the latest research. Consider supporting us on Patreon.com/PapersRead for feedback and ideas.
Twitter:
@papersreadai
Language:
English
Contact:
8958283
Website:
https://papersread.ai/
Email:
kjartan@agamecompany.com
Make Your LLM Fully Utilize the Context
Duration:00:20:48
How Far Are We to GPT-4V? Closing the Gap to Commercial Multimodal Models with Open-Source Suites
Duration:00:43:03
Dynamic Generation of Personalities with Large Language Models
Duration:00:24:33
Megalodon: Efficient LLM Pretraining and Inference with Unlimited Context Length
Duration:00:27:30
Phi-3 Technical Report: A Highly Capable Language Model Locally on Your Phone
Duration:00:15:03
Actions Speak Louder than Words: Trillion-Parameter Sequential Transducers for Generative Recommendations
Duration:00:48:19
Assisting in Writing Wikipedia-like Articles From Scratch with Large Language Models
Duration:00:35:12
Mini-Gemini: Mining the Potential of Multi-modality Vision Language Models
Duration:00:37:55
InstantMesh: Efficient 3D Mesh Generation from a Single Image with Sparse-view Large Reconstruction Models
Duration:00:20:46
From Words to Numbers: Your Large Language Model Is Secretly A Capable Regressor When Given In-Context Examples
Duration:00:36:41
AutoCodeRover: Autonomous Program Improvement
Duration:01:00:32
TrustLLM: Trustworthiness in Large Language Models
Duration:02:48:17
AniPortrait: Audio-Driven Synthesis of Photorealistic Portrait Animation
Duration:00:11:57
Fast Timing-Conditioned Latent Audio Diffusion
Duration:00:43:22
Gaussian Head Avatar: Ultra High-fidelity Head Avatar via Dynamic Gaussians
Duration:00:35:11
ReFT: Representation Finetuning for Language Models
Duration:00:33:24
Long-form factuality in large language models
Duration:00:37:15
Jamba: A Hybrid Transformer-Mamba Language Model
Duration:00:25:58
QA-LoRA: Quantization-Aware Low-Rank Adaptation of Large Language Models
Duration:00:36:22
MegaBlocks: Efficient Sparse Training with Mixture-of-Experts
Duration:00:41:52