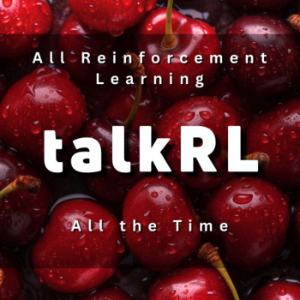
TalkRL: The Reinforcement Learning Podcast
Technology Podcasts
TalkRL podcast is All Reinforcement Learning, All the Time. In-depth interviews with brilliant people at the forefront of RL research and practice. Guests from places like MILA, OpenAI, MIT, DeepMind, Berkeley, Amii, Oxford, Google Research, Brown, Waymo, Caltech, and Vector Institute. Hosted by Robin Ranjit Singh Chauhan.
Location:
Canada
Genres:
Technology Podcasts
Description:
TalkRL podcast is All Reinforcement Learning, All the Time. In-depth interviews with brilliant people at the forefront of RL research and practice. Guests from places like MILA, OpenAI, MIT, DeepMind, Berkeley, Amii, Oxford, Google Research, Brown, Waymo, Caltech, and Vector Institute. Hosted by Robin Ranjit Singh Chauhan.
Twitter:
@talkrlpodcast
Language:
English
Contact:
6048856418
Website:
https://www.talkrl.com/
Email:
robin@pathwayi.com
Vincent Moens on TorchRL
Duration:00:40:14
Arash Ahmadian on Rethinking RLHF
Duration:00:33:30
Glen Berseth on RL Conference
Duration:00:21:38
Ian Osband
Duration:01:08:26
Sharath Chandra Raparthy
Duration:00:40:41
Pierluca D'Oro and Martin Klissarov
Duration:00:57:24
Martin Riedmiller
Duration:01:13:56
Max Schwarzer
Duration:01:10:18
Julian Togelius
Duration:00:40:04
Jakob Foerster
Duration:01:03:45
Danijar Hafner 2
Duration:00:45:21
Jeff Clune
Duration:01:11:11
Natasha Jaques 2
Duration:00:46:02
Jacob Beck and Risto Vuorio
Duration:01:07:05
John Schulman
Duration:00:44:21
Sven Mika
Duration:00:34:56
Karol Hausman and Fei Xia
Duration:01:03:09
Sai Krishna Gottipati
Duration:01:07:48
Aravind Srinivas 2
Duration:00:45:10
Rohin Shah
Duration:01:37:04